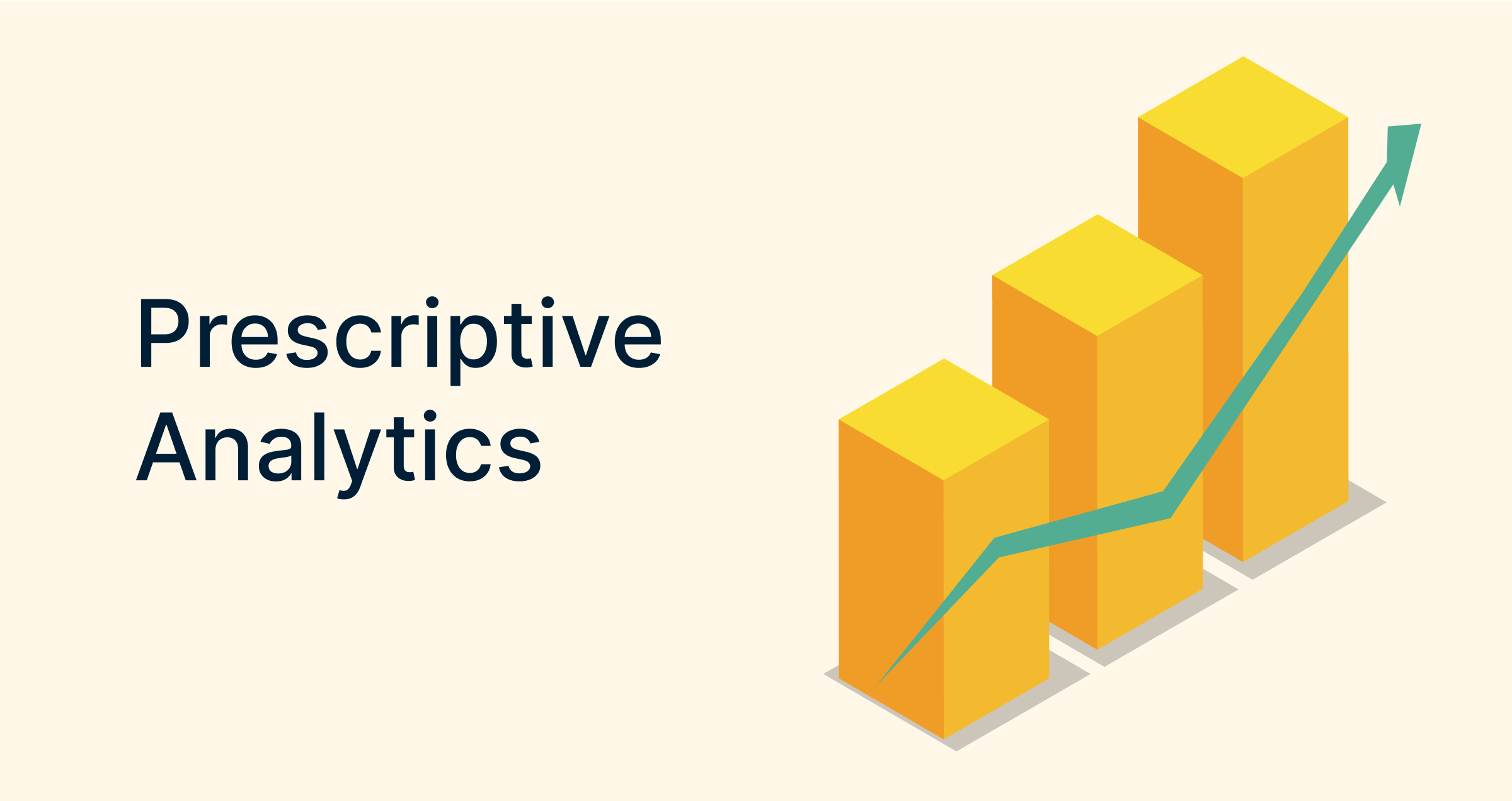
Prescriptive analytics are a high-level form of advanced data analytics that helps organizations understand and predict future outcomes and prescribe the best action to achieve desired objectives.
Essentially, when a business asks, “How do we do this?” or “How can we achieve this?”, prescriptive analytics provides the answers.
Prescriptive analytics uses large data sets, machine learning, artificial intelligence (AI), and advanced algorithms to provide actionable insights to assist decision-making and inform business operations in real time.
This in-depth examination of prescriptive analytics includes identifying what it is, how it differs from predictive analytics, and examples of its application in our day-to-day lives.
It also includes an explanation of how it works, the most commonly used tools for prescriptive analytics, and the benefits and potential challenges of organizations’ adoption of it.
What is Prescriptive Analytics?
Consider it the next level up from predictive analytics. While diagnostic analytics answers the question ‘What happened?’ and predictive analytics addresses ‘What might happen?’, prescriptive analytics answers ‘What should we do?’
Again, by considering the multiple variables that affect a business’s success, prescriptive analytics recommends precisely what an organization should do to maximize desired outcomes.
Visit this article next to explore predictive analytics in more detail.
Prescriptive Analytics vs. Predictive Analytics
Comparing prescriptive analytics with predictive analytics better highlights its role.
The latter, particularly in finance, relies on the analysis of large datasets to determine future trends. Based on historical and current data, it identifies patterns and makes forecasts for subsequent periods.
The traditional methodologies employed in predictive analytics include well-established statistical techniques. More recently, big data and machine-learning technologies have played a significant role.
Predictive models in finance are commonly applied for the following purposes:
- Budgeting
- Forecasting
- Risk management
Whereas predictive analytics helps assess what might happen in the future, prescriptive analytics takes it a step further. It uses the outputs of predictive models to inform users about what actions will help them reach their desired objectives.
For instance, if predictive analytics shows that a group of your customers is likely to churn, prescriptive analytics will tell you what measures you might take to keep them.
In this article, you will learn how to retain customers and keep your churn rate low.
How Prescriptive Analytics Works
Prescriptive analytics works by collecting vast data from multiple sources and subjecting it to sophisticated algorithms that churn out analyses.
The process generally consists of the following steps:
Data collection: The system collects data from various sources, such as historical information, real-time inputs, and third-party data sets.
Modeling and simulation: The system repeatedly trains machine learning algorithms to create models representing possible future scenarios. Each model consists of several factors and the dependencies of one factor on another.
Optimization: The system picks the best course of action by running simulations of potential actions and their consequences. This might entail solving complicated optimization problems in light of objectives and constraints that are domain-specific to the organization.
Recommendation: In the last stage, the system offers explicit instructions or suggestions about achieving the best possible outcome. These recommendations are usually designed to be easily understood and actionable by the person responsible for making the decision.
For example, prescriptive analytics in the health sector might examine patient data to recommend personalized treatment plans to improve health outcomes and reduce readmissions.
In marketing, it could advise the best timing and content for an email campaign to maximize market share.
Examples of Prescriptive Analytics
Prescriptive analytics is used in various sectors, and for good reason: each industry benefits from the ability to suggest data-derived actions.
Here are a few real-world examples:
Finance
Prescriptive analytics in finance, often applied to fraud detection, risk management, and investment decisions, uses historical transactional data to identify patterns that could signal suspicious activity and provide recommendations for preventing that activity.
It could also optimize portfolios by recommending the best investment strategies to suit the market and risk conditions.
Marketing
Prescriptive analytics can help firms better manage their clients and customers. Those firms can use their clients’ and customers’ data to suggest the best marketing approaches, such as the ideal time to deliver an email or the best messages for each particular group of customers.
Supply Chain Management
Recommend inventory levels, production schedules, and delivery routes. This can result in significant cost and time savings.
Healthcare
Hospitals and other providers use prescriptive analytics to personalize patient care.
For example, prescriptive analytics can recommend the best treatment options for an individual patient by considering patient history, current health data, and medical research. This can speed up recovery and avoid complications.
Travel Hospitality
Airlines and hotels use prescriptive analytics to engage in dynamic pricing and develop ‘buckets’ or groups of customers to whom they can target different offers.
Prescriptive analytics measure booking patterns, market conditions, and customer preferences to develop pricing schemes that maximize revenue and customer satisfaction.
How Companies Use Prescriptive Analytics
Most sectors use prescriptive analytics to improve decision-making. Here are some examples of how organizations use prescriptive analytics:
Improved operational efficiency: Information derived from multiple data sources across the business can reveal bottlenecks and inefficiencies, which prescriptive analytics can correct.
Prescriptive analytics recommends specific actions to improve and optimize processes while reducing costs and improving productivity.
Tailoring marketing campaigns: When marketers know that different slices of their audience will react favorably to different patterns of campaign delivery, they can use prescriptive analytics to optimize their campaigns.
This might involve recommendations of which channels to use to reach which audiences, when to target them, and what content should best attract them to ensure the greatest engagement and conversion.
Enhance customer experience: Prescriptive analytics can help companies tailor customer experience by analyzing customer interactions and feedback. These might include suggestions for customized product recommendations or personalized customer service.
Risk management: Prescriptive analytics can also be used to manage risk. In industries such as finance and insurance, it analyses historical data to identify risks and suggests strategies to minimize them.
This can be particularly useful in fraud detection, credit risk assessment, and compliance management.
For example, banks and other financial institutions leverage prescriptive analytics to identify and mitigate fraud. This technology analyzes transaction information and recommends actions, such as blocking suspect activity.
Pros And Cons Of Prescriptive Analytics?
Prescriptive analytics isn’t perfect—it has drawbacks and advantages. A clear assessment of these will help organizations decide whether to adopt it.
Pros:
Prescriptive thinking: Prescriptive analytics recommends courses of action, reducing the role of intuition and gut feeling. Therefore, decisions are likely to be more rational and objective.
Resource optimization: Prescriptive analytics can help your company allocate resources more effectively, whether budgeting for projects, staffing, or scheduling.
Personalization: Prescriptive analytics help businesses tailor services and products, which could encourage buyers to stay loyal to the company.
Preemptive problem-solving: Rather than ‘firefighting’ after problems have already arisen, prescriptive analytics help organizations anticipate and solve problems before they happen.
Cons:
Complexity and cost: Integrating prescriptive analytics can be expensive, requiring substantial investment in data infrastructure, computational power, and expertise.
Data quality issues: Because prescriptive analytics relies on the quality and accuracy of the input data, the recommendations can be rendered useless if the data is incomplete or inaccurate.
Requires human oversight: As sophisticated as it is, prescriptive analytics is not self-sufficient; decision-making is still required. Not everything is automatable in unpredictable or complex environments.
Resistance to change: Management or workers may resist the introduction of prescriptive analytics if it requires changing existing processes or decision-making procedures.
Best Tools for Prescriptive Analytics
These include tools to help organizations implement prescriptive analytics: This software provides the infrastructure to process data at scale, perform simulations, and provide recommendations that executives can act on.
Here are a few popular options:
Datarails’ “Insights” by Genius
Datarails recently launched ‘Insights’ by Genius, an AI engine purpose-built to automatically identify hidden business opportunities in financial planning and analysis (FP&A).
Insights alerts businesses to trends and variances and provides instant, actionable recommendations so organizations can capitalize on growth opportunities before problems become full-blown.
IBM Decision Optimization
Enabled by the broader IBM data science platform, this tool helps businesses make optimal decisions about everything from supply chains to financial planning.
SAP Integrated Business Planning
This is SAP’s version of predictive and prescriptive analytics, which it claims helps clients ‘plan their operations to match business strategy.’
Microsoft Azure Machine Learning
Azure offers a suite of tools for building prescriptive analytics models, the ability to handle large-scale data processing, and integration with other Azure services. This tool is known for its excellent data privacy features.
RapidMiner
RapidMiner Studio includes data preparation and model deployment tools for prescriptive analytics to help businesses extract insight from their data.
Conclusion: What’s Next for Prescriptive Analytics?
Prescriptive analytics can be considered the next generation of data analytics that can help organizations shape the future instead of just predicting it.
Whether it’s operations in healthcare, finance, marketing, or supply chain management, prescriptive analytics can help organizations transform how they work and make decisions.
With the right tools and implementation, it can be a powerful tool in any organization’s data analytics toolkit.
To get the most powerful data analytics on your side, request a Datarails demo today.