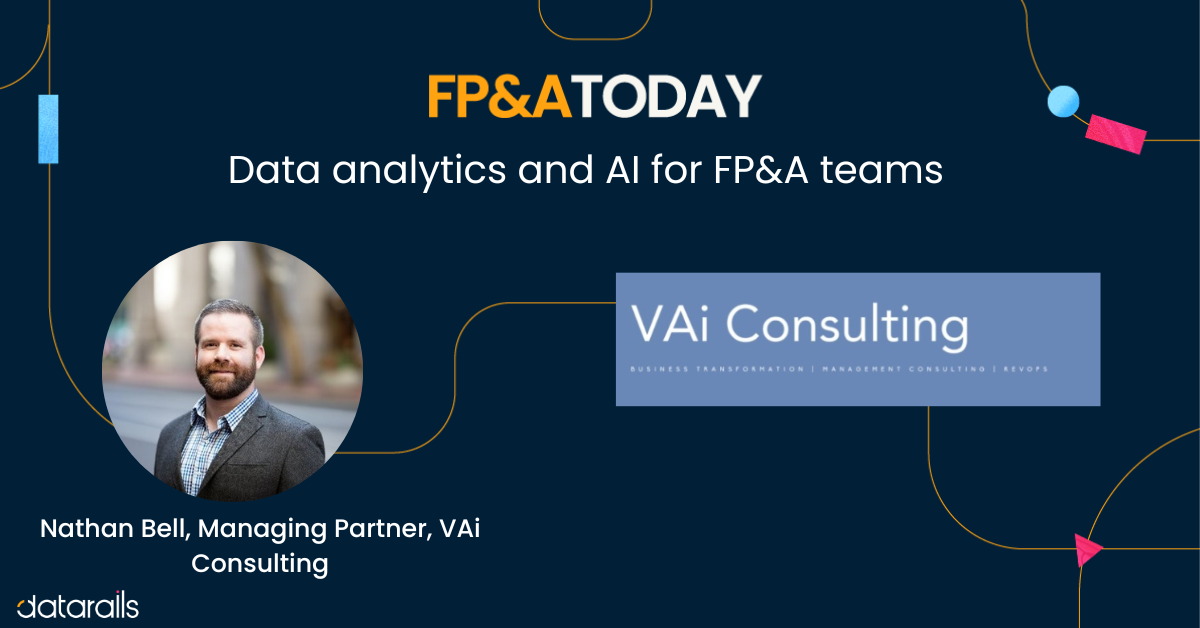
Getting FP&A teams to harness their data to reach their goals is the holy grail. Nathan Bell, Managing Partner, VAi Consulting, is among the most in-demand experts. Bell has unique experience– having started in computer science, before leading finance teams at Native American Bank, Digital Media Trends and Gartner– where he advised hundreds of FP&A teams facing chaotic data situations). In this masterclass, he shares a practical framework looking at the expectations, fear and hype surrounding data, analytics and AI for FP&A teams.
In this episode:
- From a computer degree to leading finance teams
- How Nathan discovered the power of telling stories as Chief Credit Officer at Native American Bank
- Overcoming the challenge of presenting to non- financially literate CEOs
- The challenge when finance is “last in line”
- When companies don’t have good data and what FP&A teams can do
- “Federated data governance” and avoiding turf battles
- why setting up self-service data as CFO came back to bite me
- Using a “metrics cascade” clarifying data and metrics
- Critical thinking as the new superpower for finance teams in the AI age
- Key to setting up pilot AI programs
- Dinner time when your wife is also a CFO
Key quotes: Remember PPDAC. Problem Plan, Data, Conclusion.
Your Plan, what Data you need to capture against that plan, analysis, and Conclusion.
“If you can master that in that order, I think from a skillset, you’re, you’re ready” – Nathan Bell.
Critical Thinking as the new Superpower for FP&A
“Critical thinking is gonna be more powerful than ever. I don’t think there’ll ever be a situation where we’re going to let AI make the decision without going through a human to look at the data. We need to ask what do we do? What’s the action? What’s the decision?”
Connect with Nathan Bell on Linkedin https://www.linkedin.com/in/nathan-bell-1038662/
https://vai-consulting.com/
Transcript:
Glenn Hopper:
Hey everyone, and welcome to FP&AToday. Today we are welcoming Nathan Bell to the program. Nathan is a seasoned business transformation consultant with over two decades of experience in finance and data analytics. He has served in multiple finance leadership positions, including CFO, SVP, director and board member. Throughout his career, Nathan’s focus has been on harnessing the power of data and analytics to drive growth, profitability, and efficiency. He has an impressive background, including roles as managing director of business transformation, SVP of Data and Business Analytics, and Senior Director of Data and Analytics in the finance practice at Gartner. He’s also held executive and board member positions at Digital Trends Media Group for 13 years, and served as the Chief Credit Officer at Native American Bank. With his extensive expertise in developing and implementing data and analytics strategies, establishing effective data governance policies, and leveraging advanced analytics and AI ML technologies for forecasting and scenario planning, Nathan is a true thought leader in the field of finance transformation, and today we’re really gonna dive in on the power of data and how finance leaders can leverage it to improve finance operations. Please join me in welcoming Nathan Bell to the show. Nathan, how you doing?
Nathan Bell:
Good, good. Glad to be here.
Glenn Hopper:
Yeah, glad to have you here. As a narcissistic podcast host, I, I really am excited about having you on because I feel like I’m in a lot of ways interviewing myself, but, you know, the improved version, the smarter version, of myself, <laugh>,
Nathan Bell:
I dunno about that, but yeah. Yet to be seen.
Glenn Hopper:
Yeah. <laugh>. Looking at your background thinking about your career, I mean, it has really been defined by your focus on data and analytics, and I was wondering, to start us off, can you kind of take us through your career with a specific focus on the integration of finance and analytics and, and k kind of how your experiences at Gartner Digital Trends Media Group and Native American Bank, how they shaped your perspective on the role of data and analytics and finance?
Nathan Bell:
Yeah, absolutely. I think I like to actually start at the beginning, the real beginning here, where my path ended up to, to this place I’m at now. And interesting enough, I came out of my undergrad with the business computer systems degree, and, and you’ll see how I’ve gone full circle with that. I actually started coding in Cobalt in Fortran, out of school at TransUnion, which you may be familiar with, one of the three large credit bureaus. And I was doing that for them for about a year and a half when I had my, I call it my office space moment, if you’ve seen that movie before. And he is sitting there and, you know, every day is the same. And in a sea of cubes, I was also in a sea of cubes just coding away on a parallel database and then making sure everything looked good and then pushing it live.
And about a year and a half in, I had a, what they call a six month checkup, if you will, with my boss, Linda. And she said to me, Nathan, you’re doing really good. If you keep it up in 10 years, you can have my job. And I just kind of had that moment where I’m like, okay, no, no, you know, I’m on the 10th floor of a 40th floor building, 10,000 employees. I could just see a sea of cubes when I looked up and I’m like, okay, I don’t think this is what I wanna do. Didn’t quite know what I wanted to do at that time, but ’cause I was well trained in the, in the background of credit, I actually was fortunate enough to get an opportunity to jump into commercial credit because of that training I had on the credit bureau side.
So I actually was fortunate enough to get an opportunity to spend some time about three years sharing an office with the founder and president of a company called Nationwide Funding that was all around commercial credit. In order to do that job, I had to learn how to basically underwrite, read financial statements, spread ’em out, analyze them, and then send those off to, to banks to get approval for. It was large commercial leases. At the same time, I went and got my MBA in finance, and that was my first dabble into finance, but starting with technology background. And that ultimately then led for me to, to go into commercial banking. And then from there, being a senior commercial underwriter and underwriting, I don’t know if you’re familiar with commercial banking side of things, but in corporate finance. But on that side, we were doing very large commercial real estate loans and what’s called CNI loans, which is basically operating lines of credit.
And those credit memos were depending on the size of the loan, anywhere from 50 to 60 pages long. In order to do that, you’d use some software called Moody’s. I’m familiar with that, to spread the financials out. It’s kinda something that credit analysts do in banking. Is that sound familiar? Oh, yeah, yeah, yeah, yeah. So we’d put those together and then we would present our proposal, and it was really part storytelling. I only just spreading the financials, but it was actually pulling them to life. And we’d go through what was called the five Cs of of credit in order to make a story out of those financials. And I just fell in love at that point. I just knew like forever my, my career had been changed. That was something that just for whatever reason came easy, resonated with me. The, the technology and tools I’ve always been an early adopter was no problem.
And where I excelled was taking all of that and transforming that data into a written story and narrative, and then presenting that to the board loan committee and making a case for should we do this loan? If we do, what kind of covenants? Where’s the risks? Those types of things. And that ultimately led me to that position as Chief Credit Officer at Native American Bank. I went to the, to the Graduate School of Banking at CU Boulder to help training with that. And was kinda one of those natural fits. I had a really strong mentor and coach, because I was pretty early in as an exec. I was 27 at the time, but I was so strong on that underwriting component. They took a chance on me and they thought, well, we can teach him that leadership later, we’ll bring him up on that side of things.
But he’s so good at this underwriting component, which is, if you, if you’re familiar with banking, that chief credit officer’s job is to protect the capital of the bank. And of course the bank’s depositors. And I just was fortunate enough to grow into that and then learn the whole kind of corporate governance, the corporate role, being on the board, presenting to the boards. And then that was my, again, one of my first forays into this concept of financial literacy. For me, it was easy to assume that everybody understood what I was looking at and what I was seeing holistically. And having to present that to non-financial literate people was, was a huge challenge.
Glenn Hopper:
Yeah. And that’s, I’m, I’m so glad you started off with the, the narrative part of it and the storytelling, because that is so much, and it’s so <laugh>. Interestingly, my career, I started out as a journalist, and I think that I got a solid foundation in telling stories and constructing a narrative around that. So kind of like you, when I came to finance, it was, if you can tell the story and you’ve got the data to back it up, then it just makes it that much more compelling. And I think certainly in my career, it helped, helped me to move forward, is just making sense of these financial statements that maybe the rest of the management team, you know, they, they don’t look at financial statements all day like we do. So being able to tell them what it means for them, and whether it’s through KPIs and, and metrics, or if it’s just making sense of what EBITDA means compared to cashflow, that kind of stuff. So tell me a little bit about some ways that you’ve seen through your career that telling that story has been a really important part of, you know, first you, you aggregate the data and you start to make sense of it and, and, and figure out what it means. But then you’ve gotta convey that to an audience, whether it’s the CEO or the board or investors or whoever it is. Do you have some good examples of how you’ve used data to tell stories in in previous positions? Yeah,
Nathan Bell:
Absolutely. I think I, I cut my chops for sure within that commercial credit role, because you really can’t succeed or, or make it to the top there from a credit perspective if you can’t tell the story, it doesn’t matter how smart you are in here for you to understand, we should make this song or not make this song. It doesn’t matter if you can’t convince the rest of the, the team that this is good for the bank or bad for the bank and, and those types of things. And I, I think being at Native American bank, and then I’ll get to the rest of my career, but what was more compelling too, that was important for that part of my kind of story and journey is that they were different from a commercial bank perspective. The American Bank had a very strong mission and vision, almost a nonprofit mission vision, if you will.
It was capitalized by 12 tribes, federally recognized tribes with the mission to bring capital to Indian country. And that brought some, some unique lending circumstances. So even more important to be able to get that story across when you’re making loans and sovereign nations, if you will. And with a kind of a mission driven, where the point is to obviously bring capital to, to the Native Americans and Native American owned businesses. I think honing that kind of storytelling was really important. Some of those loans were critical to those communities, and you needed to get it by, we were OCC chartered and regulated, so we still had to comply. We had constant probably every six, 12 months audits we had to go through. And I needed to also tell the story to the auditors as well. The OCC auditors when they came in, wanted to understand why this loan doesn’t look the same as what they’re used to seeing over here. But yeah, in order to deliver on that mission and vision for an American bank, it was critical to learn how to tell that story.
Glenn Hopper:
Anyone who’s worked in finance for a while or worked with data for a while, it’s easy to go back to what is it? The Mark Twain quote about lies, damn lies and statistics. And it’s, and I think Malcolm Gladwell even talks about it where you can, I love it. You know, you slice and dice and, and, and when you talk about data visualizations, you can talk about ways you could mislead by changing the Y axis, you know, whatever the range is or things to that. I think that, you know, one of the issues that I see a lot is when you come into a company and are looking at their financials and you start asking questions, you know, in, in immature companies, I’ve, I’ve spent most of my career in the startup world, you start to see how how these particularly young businesses, but I’ve, I’ve seen it in in public companies as well, where they don’t have a good handle on the, the data. So it’s hard, it’s hard to construct a narrative if you don’t have your data sorted out. So I guess thinking about, you know, you and I both share this passion for helping finance teams harness the power of data and analytics to achieve their goals. <Laugh>, what are some practical examples of how using data and analytics has transformed some of the finance teams you’ve worked with?
Nathan Bell:
Again, going back to my commercial banking days, when you’re trying to put a story together and you’re trying to help ’em get that loan, and you’re working with these companies, finance teams and trying to get the data from them in order to, to craft your story, I was fortunate enough to help them in a lot of ways, and you’ve probably done this too, and I think you mentioned this before, is the moment you start porting that data over and you start looking at this chart of accounts and you realize that these, this data’s not structured in the way, that’s just gonna be an easy port into the software that you’re using to make that underwrite. And then ultimately, that role ends up becoming more of a consulting and you’re like, look, I can’t even help you get this loan until we sit down and figure out how to organize your GL and your chart of accounts in such a way that we can capture the right data to tell the story We need to tell to the board to get your loan approved.
So there was that for sure. And then after the Native American Bank days, I was fortunate enough to get an opportunity to go for startup, actually, media. You were in journalism, I was in Media, digital Trends, media Group. I was CFO there as employee number two in the, in the company. It was, it was quite a ride and learned a lot there. Drew from, you know, three of us to 500 plus employees include contractors. But I had an opportunity then seeing what I saw up to that point of, of how crazy the, the structure was from a data standpoint in these other companies I worked with. I had a chance to do it from scratch at digital trends, literally from scratch, from picking the tools, from setting up the GL to figuring out what kind of data I would have wanted to see if I was pulling this out into reports.
And then also dealing with the two co-founders who had zero financial literacy and, and frankly, I, no offense to them, didn’t have any interest really, either in <laugh> learning that discipline. So the onus was even more on me to figure out ways to not just get the right data, but then start structuring that and reporting it to them. Especially as we were growing, we were raising capital. We did a Series A, went down to the Bay Area, did the whole pitch, you know, to Excel and Sequoia and all that. And of course that came with a lot of oversight and requirements from a reporting standpoint too, as you’re probably familiar with the VC world.
Glenn Hopper:
Yeah. So you were, you were employee number two CFO, and you came in and you had the, the other founders were not, not as interested in, in the data as you were, but you managed to establish a data governance committee and you launched a proprietary first party data platform. Tell me about some of the insights and kind of how you walked through and set those up and what that meant and what kind of the before and after of establishing all that.
Nathan Bell:
Yeah, we did, it was, it was a long journey to get there, and a lot of it was out of necessity. Some of it was, if you’re familiar with the nature of digital media, you have real time data coming at you. So a lot of it was, as I used to just say to my clients at Gartner you have financial data and then you have performance and operating data and metrics, which is kind of the convergence of financial data with non-financial data, whether it’s a CRM or marketing platform. In our case, it was traffic to the site and it was performance of ads. And as we were there, my team, you know, as I grew and grew that team, it was me originally just trying to get what I could outta QuickBooks. And then of course, using Excel heavily, then making that migration to, to NetSuite from QuickBooks.
And the more and more I was putting this data together and starting to give insights to the team. It was the editorial team, it was the marketing team, it was the e-commerce team. ’cause We were a extreme electronic review site. Everybody got more thirsty, as you can imagine. They started getting information that they weren’t used to getting, and it was presented to them in a way that wasn’t so finance, if you will. My team ultimately realized we weren’t gonna be able to give them access to the system themselves. ’cause There was a lot of hunger for I need this, I need this faster, and I need to be able to manipulate it myself rather than wait for you and your analysts to put this together. So we went out, you can imagine on this journey, we went out and got a BI tool.
And for us, it was originally Tableau. And then we stood up. I had, it was fortunate enough, we had a dev team that was an AWS shop. So we stood up a Redshift as our data warehouse and then pointed NetSuite into there, connected. We were using Google Analytics for all of our data for, for the editors, and then put those two together. Those were the two that we started with originally. And that was more than enough for me and my team to, to really spend the next year just kind of putting that information together and putting together workshops too, with the different departments and teams trying to figure out, you know, one of those blank canvas exercises. Hey, if you got everything you really wanted to do your job, what would that look like? I always start with the realm of like all possibilities. I don’t want to limit them and tell them, oh, we can’t do that out of the gate, just because we don’t know how to, or the bi tool is, is not appropriate. So we kind of started with that. I, I like to say it kind of like this promised land, if we started there and then work backwards,
Glenn Hopper:
Fp and a today is brought to you by Data Rails. The world’s number one fp and a solution data rails is the artificial intelligence powered financial planning and analysis platform built for Excel users. That’s right, you can stay in Excel, but instead of facing hell for every budget month end close or forecast, you can enjoy a paradise of data consolidation, advanced visualization reporting and AI capabilities, plus game changing insights, giving you instant answers and your story created in seconds. Find out why more than a thousand finance teams use data Rails to uncover their company’s real story. Don’t replace Excel, embrace Excel, learn more@datarails.com.
Yeah, so you and I, I mean I think we both a approach data and finance as being, you know, o opposite sides of the same coin. But I think a lot of people, and you may see this in your consulting, you know, finance is a customer of the data, not, they don’t own it, you know, obviously GL Finance has, but getting the data from others, and I’m, I’ve pushed for years in, in, in companies where I’ve served A CFO that it’s, I want to, I don’t need to own <laugh> the data.
I need full access to it. And I, I’m not gonna, maybe I don’t own the data, but I’m gonna be heavily involved in sort of developing the data lexicon and understanding what the source of truth is. What would your advice be? ’cause I think you and I could go down this rabbit hole where we’re spending just too much time really on the, the data science part of it. And I think about our audience. I think for finance people who maybe don’t have an advanced data strategy right now, or, or maybe you’re not big enough to sort of have embedded FP&A people across teams. What would your advice be, or how would you go about in a, in a company where, you know, maybe there are different sort of organizational structures where finance and accounting are, we are just, you know, we’re the GL and the financial statements and those reports, but we know, you know, the, the metrics that we’re gonna track and, and kind of identifying the levers and for us to be better at forecasting that we need access to the data. Have you seen any examples of companies where you’re coming in and you’re having to overcome this sort of, we’ll call it an old school mentality of getting access to the data and how finance should work with it and, and other groups to, to have a common data dictionary, lexicon, sources of truth, all that?
Nathan Bell:
Absolutely. Honestly, it was probably the most frequent scenario that I saw when I was advising at Gartner and then consulting as well. And fortunately I went through that same friction at my own organization at Digital Trends. As you know, going back to that journey, we stood up a BI tool Tableau, and we had, it had to support our, our data warehouse originally and having to work back and forth with those folks and figuring out what we needed. And in fact, if you can imagine, there was a lot of battle between the CTO and me originally as far as, as we continued to grow that team. And I wanted to go from an fp and a to a true advanced analytics syndrome excellence. What kind of fp NR two, FP and a to advanced analytics. If you kind of go that evolution, there was that battle with the CTO of where are those resources set, who should control it?
And anytime they see something that says data or engineer in a function or title, the CIO or CTO typically is thinking, that’s mine. That’s my world. And you’ll have to submit a ticket if you need to get access to something or create a new pipeline, or even if you want a new table or field added, it depends who’s administrating, you know, whatever system you’re connecting to, whether it’s the CRM or, or marketing. I’m very passionate about this. I wrote about this at Gartner and have a, a few strong-minded opinions in my papers. They’re about finance, taking more of a leadership and, and kind of getting the front of a line in front of this, because the hundreds of clients I had at Gartner that constantly came up the CFO and the finance team having this barrier, this obstacle where they couldn’t get what they needed for, especially the FP&A, it was always typically the FP&A folks, they couldn’t get what they needed because it was dragging their feet.
It didn’t understand what they were asking for. It gave it to ’em and said, here, here’s everything you need. And then just walked away, right? And then FP&A leaders are like, this is great, but I’m missing half of what I need. And then having to get back in line with it for the, you know, priority wise. And it actually goes back to why I firm believer, for folks that are listening to this that are trying to think about moving to true advanced analytics and FP&A leading that you do need your own resources. But in order to kind of, I would say smooth over those types of conversations requests, you need to set up data strategy enterprise wide. You need to set up a data governance, not just within IT, but within the organization. That should be tied to your data strategy. The CFO or head of FP&A should be on that.
The way I recommend structuring that kind of thing is the CFO sits at the kind of council level on that group working with the board, and ideally a couple of other execs. And then they have a committee below them where you would have a representative from every major stakeholder. So FP&A typically I recommend be the leader on that level below the, the CFO. ’cause They’re kind of day-to-Day operational. They understand it. And a lot of times with the CFOs, they’re, they’re at a point where they have so much other things to do. They’re not gonna be focusing on data literacy or advanced analytics. They want the outcomes, right, of predictive and prescriptive analytics forecasting. But really that FP&A leader needs to sit on that committee and work with their IT counterparts and other business unit owners like heads of sales and marketing.
And that’s where you kind of get that holistic kind of teamwork, if you will. Those folks are the ones that own the metrics and KPIs that we’re tracking. And then I, I don’t want to go off to a rabbit hole, but it goes into this concept of assigning data stewardship and then ultimately data ownership within that hierarchy. So everybody understands kind of their role within, to your point of that data, like most folks are creating it, not finance. What are those rules that are governing that? And you, if you don’t have that committee and council above it, as you can imagine, it’s just total chaos. It’s multiple versions of truth everywhere. Hearing
Glenn Hopper:
You talk about that, I’m thinking about in my career, going back to, I mean, what always would happen is, you know, your back of the house finance and accounting is asking for something from IT. Well, you’re never gonna get prioritized over revenue producing, you know, customer facing product product development. So you’re always in the back of the line, you know what you want. So it leads to, or at least historically has in my case, you know, citizen development, which was so danger, like going back to <laugh> early two thousands, where like where I’m running a, a massive access database on my <laugh> work laptop, you know, that that really is just, you know, duplicating DA data and it’s just a, it was kind of a mess or you know, get trying to hack your way into access to things in, in the company.
But here’s, here’s the problem though. I mean you know, I think your background is unique with starting out in computer science and then moving into finance later. That’s not what, that’s not the path that most people take. So we know in FP&A that we want to own this data, or at least have unfettered access to it and, and being able to define this, you know, sit on the council, but we don’t have the skillset to manage the people under it. So how do you picture, like what’s the best way to set up a team that you can have, you know, your own resources for the sort of the technical part of it, but then also the domain experts in finance and accounting that can understand it and help shape all this and, and really build the products that you need. On the finance side,
Nathan Bell:
I think what you’re talking about here, at least how I’m hearing what you’re saying is, is something that I’m a big believer too, is this idea of federated adaptive governance, data governance. And what that is, is that avoiding these kind of turf battles and silos amongst folks is doing this, not just data mapping, but mapping the decision makers. I like to do kind of a raci, if you will, against those types of things. Once you map those metrics and those KPIs, you understand who owns them, who’s creating them, who administrates the system that those are being created in. And you assign clear owners and stewards. Stewards are typically managers, business unit owners that understand their responsibility. Again, all of that’s rolling up but this idea that you’re trying to force the organization to a single version of truth will always fail, right? You’re trying to avoid the multiple versions of truth.
And I like to tell this story where I was presenting and, and this will kind of go circle back to I think what you’re talking about. I was presenting at an exec offsite, the board pack, the quarterly board pack, and I had the finance data and I went first. Now this, mind you, this had already gone out. The quarterly board pack had already gone out to the shareholders and the board, I presented my portion first, and then after I presented the head of sales, did their part, the head of marketing, product, hr, you know, as the day went, each person came up and said, I saw what Nathan presented, but my story’s a little different. The data I’m about to show you is similar, but I’ve removed this thing, added this thing, you know, and changed this narrative. So as the day went by the CEO and the COO and some board members realized they were seeing so many versions of the truth, at the end of that day, the CEO pulled me aside and said, Nathan, I’m absolutely paralyzed right now.
I have no idea which direction we should be going right now. ’cause I just got three, four different presentations of how we’re performing. You’re supposed to be the version of truth I’m listening to as CFO putting together with the FP&A team, those financials that go out the door, only to be told after the fact from my other heads that that wasn’t quite true. They had a different version of it. So at that point he said, this can’t happen again. This has to be the last quarter that we do this. What is the solution there and how do we get everybody aligned on that? Going back to your like, finance doesn’t own it, we report it situation. And clearly each department had had a version that the story they wanted to tell that they didn’t think finance was doing appropriately on their behalf, right? Which was a lot of the cause of this shadow metrics at o KPIs that was going on within the organization.
Glenn Hopper:
My argument has always been, look, if finance is trusted to be the unbiased reporter of the truth of financial statements, we should also own the rest of the metrics because we’re not gonna come up with, well, this quarter we’re gonna use this, but this quarter we kind of missed our mark, so we’re gonna redefine it to make our department look better. It’s like we’re just the across the board, I just hit my mic there, but the, we’re just across the board. You know, we’re, we don’t have a dog in this fight. We’re just the reporters of it. And you know, that’s, that’s been my approach to, to metrics through the years is to get to everybody agree upon how we’re gonna define this. And that doesn’t change unless something fundamentally changes below it. But you know,
Nathan Bell:
<Laugh> right?
Until they feel like it needs to change. Because to your point, it’s an incentive alignment issue. Their incentives in sales and marketing and product are not necessarily aligned to what finance is reporting, right? Right. It doesn’t serve their interests. So they might agree with it in that moment. Like, yeah, this is what we should be tracking and reporting until it shows them, to your point, not in a fair light or not hitting the commission or bonus that they, that they want to hit. And I think what happened, I, I kind of shot myself in the foot on, on this story here too, because we were pushing so hard on the team to roll out self-service analytics. So we pushed Tableau out to the organization. So this really came back and, and really bit me in that meeting because when asked each head of, you know, the departments that were reporting slightly different numbers where they got their data, they all said, we got it from Nathan’s Tableau, the reports, he stood up for us. And that just kept me, I just kept cringing as, as the day kept going. I just kept, you know, one of those face palm moments and I’m like, what have I done?
Glenn Hopper:
Yeah. And that’s, so that’s an interesting thing because I’ve always argued for, I love self-serve reports, and I know you’ve written about this and I, I, I do wanna talk about it, but I, so the plus side to me is when you decentralize the data, and obviously I’m not talking about proprietary information or whatever, but when everybody has access to the same sort of metrics and, and data and is able to figure things out, then it, it pushes out, you know, if you have access to the information, maybe your frontline employees have an insight that, that you wouldn’t have. So it’s in, in theory, it’s a great thing that everybody can access this data and figure things out. Like figure out, oh, we’re, you know, it takes us 45 days to provision the service and based on this data, here’s what I noticed. And, you know sort of bottom up process improvements could come out of it. But the flip side of that is mm-hmm, <affirmative> when everybody can get access to the data and build all their own customer reports, you’re gonna end up with these metrics that you no one else has discussed and you haven’t defined. I mean, what do you, is there a recommended approach that, that you advise on?
Nathan Bell:
That? Yes, and I’m still an advocate for self-service. At the end of the day, what you don’t wanna find your team, especially if you have a smaller FP&A team trying to support a large organization, you don’t want your team buried in tickets for report requests where they’re spending all of their time just building instead of analyzing and recommending, right. Actionable insights. And I’ve actually seen this happen at hundreds of the clients that I was working with, where they didn’t want to kind of unleash the genie outta the bottle, if you will, by doing self-service. And instead they buried their team in so many report requests every time somebody wanted to change one little thing in a report. And then it was just this backlog, if you will, of, you know, getting to it. And then the other, the flip side of that, those execs are saying in those meetings, we don’t have access.
Nathan’s team isn’t getting, you know, these updates in time. We’re running a month behind on, on the data we need to make these decisions, you know, more real time. So you’re kind of like stuck on either end of it. So what I think was more important was, and then going back to that data governance is getting everybody agreed upon, here’s self-service, but here’s the rules of the game. When you are presenting to, say, if it’s just a report that your team’s using to do their day-to-day from an operational standpoint, okay, we, we will be okay if that’s not exactly the same as what the board’s seeing and coming up with those layers of what is okay to go out the door and be shipped to this audience. So we, with that group, that data governance committee that oversees the KPIs and metrics, you agree, if this is going to the board, this is how we’re defining it within your data dictionary and glossary, this is what it has to look like.
’cause On the converse side, the board and exec team can’t remember five versions of the same metric. That’s absurd for them to have to figure that out, right? They just need the highest level distilled to them. So on the flip side, they just need a, you know, a set of 10 or 12, and then you can kind of roll that down, if you will, waterfall down to the individual needs, down to the individuals within the business units and kind of with that governance structure. So I think I mentioned this and on another call where if somebody wanted to make a change and, and say the head of sales is frustrated, and they said, you know what? This is just not representing how we’re doing right now, and the forecast Nathan is showing, I just, I don’t think that’s, it’s an underestimate for what I think it is.
And then you, you go back and you say, okay, what would you like it to show and why? So they propose that to the committee. So the whole organization gets to see instead of a silo, gets to see sales would like to change this KPI metric that is going out the door from the FP&A team. Okay, great, let’s talk about it. Talk about why they want it changed from where it’s at now, instead of them going off on their own and just doing what they want and then presenting it without anybody knowing, you know, catching you off guard in that meeting. They have to go through that process where everybody gets to see what they’re doing and why they think it should change. And then in some cases, it’s not as easy as just changing it, it’s, oh, we’re gonna have to create another data pipeline, or we’re gonna have to go get that, that information that you’ve just wanted this, you know, this new metric or this change to make. And then what you’re trying to avoid too, in that situation is folks that are trying to report on a specific KPI or metric, but because they can’t access the data, they’re doing something by proxy, they’re figuring some other type of, of KPI, that’s really not the truth because they’re frustrated that they can’t get to the right data. Again, this committee, this, this governance should, should have that process in place for folks to, to figure that out.
Glenn Hopper:
People doing some feature engineering on their own. <Laugh> coming out you <laugh>. Yeah, you’ve talked about the metrics cascade before. Does this all kind of fit into that? And maybe if you could explain the concept and provide a couple of actionable steps for our audience on the, on the metrics cascade?
Nathan Bell:
Absolutely. So the metrics cascade is something that I worked with and kind of created and altered when I was at Gartner. And it was something that came about from, you know, I think it was like a hundred plus calls in with CFOs and FPA leaders when I realized that there was kind of a, a jargon lexicon problem going on. And one of the issues was when they’re putting requests in with it, it was giving them what they thought they needed versus what, you know, finance. And then you had that barrier of, of the, of the lingo and, and literacy. And I thought, well, what if we can all agree using a framework, finance understands and, and most senior management and leaders should understand from a metric KPI standpoint using that to create the map and prioritize the work that maybe it needs to get to for FP&A to properly report.
So in order to do that and make sure that you’re not going off into a proliferation of KPIs and metrics, like most companies probably have when you’ve got like 200 reports when you log into the what you do is you force the from top down the organization to align what are the shareholder outcomes. We’re trying to get two to three of those. What are the business drivers behind those? Let’s figure that out. So you’re kind of aligning the board with the c-suite and now with the senior managers. And then once we’ve got that mapped out, what are the KPIs that are gonna be proper for us to track against those business drivers? And, you know, we’re trying to identify those levers and dials that the leaders, the stakeholders have to manipulate to get to their goals. And then ultimately the, the metrics that will drive that tracking about how we are or we’re doing against those KPIs.
Once that is laid out and everybody agrees this is it, then you can imagine the next step would be, okay, what do we need from a data ingredient standpoint to properly report on those metrics? And I think I’ve mentioned this before, but not all, not all KPIs are metrics, but all metrics are KPIs that conversation too, and helping folks figure out the differences. But once you have this map, you can color code it to a standard that you create internally. Now there’s some, some guides out there as far as the data quality standard, and again, goes back to the audience. You might have a really high standard for a board report versus just a day-to-day operating report. What does the data cleanliness need to be? And then you color code it. And once you do that, you’ve got a nice visual that finance understands and FP&A specifically owns, right?
The KPI and metrics reporting and dashboards, but also something that it can understand and the rest of the organization and, and stakeholders can understand as far as what it means to them, right? Personalizing it, contextualizing it. And then when you put a request in now to IT, they understand why this is urgent. If it’s one of those key business drivers, and this is a KPI that’s sitting at red because the data quality is is just garbage, and you put that request into it, then IT, when they’re doing their backlog grooming, right, they can prioritize it. They say, oh, shoot, this maps to that cascade. This is something that c-suite and the board is using to drive the direction of the organization, and this is sitting at red. Whoa, we need to move this quickly. Yeah,
Glenn Hopper:
That’s great. And that makes a, makes a lot of sense. And that way, that way if there are these one-off reports that are somehow valuable at a working level, fine use them. Do that. And then just know what these are the all agreed upon vetted, been through the council, these are the, the true metrics on how, you know, these are the, maybe these are more the levers that we pull that are gonna in influence the business. And then
Nathan Bell:
Kind of, i, I like to spend most of my time thinking and, and writing and talking about is advanced analytics and where that metrics cascade and that data quality ranking, where that leads to is a map to advanced analytics that gives you the direction that you need kind of that inventory, if you will, of the state of the union. Are we anywhere close to being able to automate anything, deploying any kind of AI or training the data to get to predictive and prescriptive analytics? And again, that metrics cascade that’s now gone through the rigor of a data quality analysis and color coding is gonna become apparent with the ones that are green, that, hey, these are the ones we should start with now
Glenn Hopper:
Because we are <laugh>, we’re such data geeks, I feel like we could go down this road forever. But I do want to get to, and we, and I, I, I do want to keep this from being a three hour long you know, Joe Rogan, ESKA <laugh>, well, maybe not Joe Rogan, ESKA three hour long podcast. Anyway, <laugh>. But yeah, I do want to hit a couple of other areas before we run out of time. And so we’re talking, you know, a lot about data and analytics and I’m thinking about pure finance people, and I think now, I mean, you’re seeing now there are MBAs with combined with business and analytics combined into a, a master’s program. And I’m thinking about a, a lot more people are going down that road, but for people who are working in FP&A today, everybody sees that we’re increasingly relying on data and analytics to do our jobs. But what do you, what skills do you think for someone that doesn’t have that computer science background, they’re an FP&A person, what skills do they need to start working on honing and, and picking up and how can they acquire these skills?
Nathan Bell:
Absolutely. And that’s, that’s really the question, right everybody should be asking themselves. I think, you know, finance folks, FP&A tends to be way more tech forward. So I I, in my experience talking with them, it’s a little bit more natural for them. It’s a data literacy, it’s an AI literacy kind of, I would say training and holistic strategy of how it can be used and deployed. Now, we talk about specific skills. I tend to advise and avoid folks to get really good at one particular platform or vendor. I think when you think of folks that are listening to this that are, are thinking about wanting to put some of their team members through specific training, wanting to level them up, and I highly recommend you’re doing that. In fact, you should have a whole program internally thought through about how you’re bringing those people along on that journey.
But what happens is if you give vendor lock and you have folks that are, Hey, I’m only good at Power BI, the moment somebody makes that switch to Tableau or Looker or you name it, Click or Domo, they’re lost again, right? They don’t really understand how it was put together and why it was structured the way it was. So I like for finance folks and FP&Afolks that are kinda the power users of these tools to not get super hung up on the particular tool itself. Now it’s okay to go out and get certified right in in these things. It’s important if that’s your organization is committed to, to certain tech stack. But it goes back to that critical thinking, how I can use data, what data do I need, how I can use it, how it’s going to affect and, and impact the folks that I’m working with. How to create that data storytelling skillset. Those are the things that are transferable no matter what vendor or tool you’re using, right?
Glenn Hopper:
That’s so key that, I mean the just understanding the foundation of it because when you do, whether you know, there’s a bunch of like drag and drop ai, you know, machine learning tools out there.
Nathan Bell:
Low Code, no code. Yeah,
Glenn Hopper:
Yeah, yeah. And I mean, those are great, but it’s, if you don’t understand <laugh>, the, the, the foundation of, you know, you’re, you’re trying to do something predictive and you don’t know whether to use, you know, a clustering algorithm or a random forest, you know, like you, it’s just like if you’re picking the wrong model and you don’t understand that foundational, it doesn’t matter how slick or how easy the tool is to use, or if that tool goes away and is replaced by something else, suddenly, you know, you, you’ve learned the wrong thing. <Laugh>,
Nathan Bell:
Are you familiar with the PPDAC acronym, the model? I think it was something that it actually wanted to get to as far as publishing when I was at Gartner, and I introduced it to a lot of my clients. It’s just how you go about tackling a problem with data. And it’s P-P-D-A-C is the acronym. The first P is the problem. You’re stating the problem that you’re trying to solve. The second piece, the plan, how do you tackle the plan as far as, you know, based on the problem you’re solving. The D is obviously what data now do you need to capture and gather against that plan that you’ve created in that problem. And then the A is the analysis, right? You’re gonna analyze the data and the C is the conclusion. And if you can master that in that order, I think from a skillset, you’re, you’re ready.
Glenn Hopper:
I’m gonna switch gears again one more time because this is a subject that’s near and dear to my heart, and I’m just, when I talk to everybody, I wanna see what they’re seeing, especially you with your data science background. Looking ahead, you know, some of us geeks have been using machine learning algorithms for our, our forecast for years and just you know, thinking we had this secret, magic formula that most people didn’t have. But now with generative AI, it’s, I mean, everybody’s talking about it and people who never understood it before are interested. And there’s obviously billions of dollars being poured in, into investing and building out these large ever bigger large language models. And, and the power just, it seems like every week there’s some new breakthrough, but looking at FP&A teams and knowing what it takes, the metrics, the financial statements, the forecasts, what do you see right now are the biggest opportunities and challenges, I guess, for finance teams to be able to leverage data analytics and AI to, to drive business value in this kind of, this new landscape that’s rapidly <laugh> accelerating?
Nathan Bell:
That’s the real question, right? What does this all mean for us in the finance and FP&A roles? I think as you pointed out, as, as we keep going AI , typically back in the day, at least my team and what I saw out there, in order to be really good, you had to be able to code in Python and SQL, you know, in order to create dashboards. Now it’s slow code, no code, right? AI is doing a lot of that, if you will. Now it’s more kind of engineering and architecture and thinking about this whole thing holistically and ideally deploying, I think natural language query within your, your BI tools and, and those types of things. But one of the things that I think keeps happening, and you know, I’m AI forward as you are, and you know, a lot of times I, I joke, it’s really how good you are at prompt engineering as far as what you can get out of these tools.
And I think that’s definitely a skillset set FP&A folks need to, to learn if they’re going to master these things. But too often I hear from, again, I’m kind of representing the, the voice of the exec team that is being asked to invest in these technologies, say FP&A saying, Hey, I really want this new tool. It’s really cool, it’s gonna help us. And nothing really happens. The ROI isn’t there. Maybe it makes their job a little bit easier, but the non-financial literate and data literate folks in the organization are saying, cool, we gave it to you. Now what are, we’re expecting some sort of kind of amazing insight or direction, if you will, for the organization that wasn’t there before where we were just barely getting the reports out the door. Now we’re analyzing and partnering with AI to, if you will, come up with more deeper insights or as Michael Tushman might say, opportunity gaps, performance gaps in the organization.
Using AI is great. It’s gonna help your job a lot quicker, faster, but really at the end of the day, I, I, I think you’re gonna need that analysis, that brain that figures out, okay, AI has helped me get this far. Now I’ve got this way and more extra time in the month, instead of just trying to get the close done and the reports out. What am I gonna do? What questions am I gonna ask yet? And then again, I think one of the things too is just what data do I need to capture? How clean is that data need to be for me to effectively deploy the AI tools that I have?
I definitely, definitely recommend little shameless plug for you folks, take your course on LinkedIn as well, as far as how to use generat AI for, for finance. But when I talk to all these finance leaders, it just, to them it’s overwhelming. It’s like trying to drink the ocean. They’re like, I just don’t understand how, what does it mean to me? How’s it gonna make my day today any better? And I think trying to narrow down and picking a few things in your role that AI can make a huge impact immediately would be a good start.
Glenn Hopper:
I use this quote all the time because I love it, and I think it talks to me, I can just visualize it as trying to stay one, he one step ahead of the just increasingly automated, increasingly you know, machine driven nature of, of what we do. And there’s a, a quote by Clifford Stoll, and it is data is not information, information is not knowledge, knowledge is not understanding and understanding is not wisdom. So what I see in this, as AI gets more and more powerful, well turning, you know, just the raw fire hose of data into information that’s like the lowest level, that’s the base of the pyramid. So then you, you know, what we’ve historically done is take this information, turn it into something meaningful, making it knowledge, and then once you but, but even now with new tools that are out there, and I’m not even talking AI, I’m just talking about through SaaS tools that you can get off the shelf and just, you know, you’re getting more and more knowledge as you and, and through machine learning algorithms, certainly. But so for us to stay ahead of the machines, we have to be providing a higher and higher level of value on that pyramid. So that means we need to become better domain experts, understand the, the business more understand, I mean, you know, as lower level stuff keeps getting taken in away by automation, we’ve got <laugh>, it squeezes us towards the top. So we have to stay on, on top of education research.
Nathan Bell:
That’s right. Critical
Glenn Hopper:
Thinking. Yeah.
Nathan Bell:
You, you’re spot on with that too. If you’re data entry clerk, I think you’re definitely in trouble right now. But, you know, and and fortunately AP and AR type clerks are probably, yeah, more at that bottom of that pyramid that are gonna be replaced. But critical thinking is gonna be more powerful than ever. Now, if you, if that’s a skill that you don’t have, you should be working on figuring out how to do that and interpreting the results and the data that AI is going to be giving to you very fast, the velocity is gonna increase there. And what we’ll always have that I don’t think AI can ultimately replace, is experiencing context that comes with that experience, that wisdom, right? I don’t think there’ll ever be a situation where we’re, at least maybe in my lifetime, maybe not, where we’re gonna actually let AI make the decision without going through a human to look at the data and saying, yes, this is what we should do. Making a recommendation, yes, but never just truly automating straight to the decision. So I think there that gap at the top of that, you know, that pyramid, that gap was where folks in FP&A and and finance is gonna live more and more. That what now what do we do? What, what’s the action? What’s the decision? Because we’re never gonna trust AI to fully automate decision making.
Glenn Hopper:
I dunno, I for one, welcome our robot overlords. I <laugh>, <laugh>. No, I am a, a huge proponent of, of human in the loop. And I you know, as rapidly as everything’s accelerating, it’ll be I hope that at least for the remainder of my career that we <laugh> that we’re still needed. So just trying to find ways to add that value.
Nathan Bell:
I also wanna say too, you know, highly recommend folks that are listening to this, set up some pilot AI programs within the finance office and stand up some citizen data scientist programs and skill training and just start playing around with it. Obviously start at noncritical things that are, you know, if you make the wrong decision or, or insight, it’s not gonna derail the company, but there’s no better way than just getting into it, as you know, and
Glenn Hopper:
Great advice. So, and that could be a whole other episode as you know, we could go through that. I, I think I’m sure you saw the, it’s probably been around a couple years, but it was the Harvard Business Review. It was a two by two matrix on how to select which projects to go after with Gen AI. And it was on, yes, I think the y axis was demand, and then the X axis was risk and but figuring that out, but finding these low stakes, something to play around with. And that’s, I advise that all the time. Get out there, just find something small, try it out. So, so that you at least understand what’s how the tools work.
Nathan Bell:
I like to couple that with Jeff Bezos’s decision matrix, right? It’s one of those, if it’s, is it a one-way door? Is it a two-way door, a decision we can make quick and easy and is it reversible? Kind of overlay that into kind of those pilots as well, right? Like what’s the worst that happens if this thing goes live and, you know, can we reverse it quickly? Yeah. Okay. Let’s do it.
Glenn Hopper:
We try to end the show on, on two questions and one of ’em is probably gonna sound very archaic to you, but I’m gonna ask it anyway. But first off, we just dove straight into the, our careers and, and data and FP&A What is something that not many people know about you that you’re, you’re willing to confess to to the FP&A Today listeners? Oh, sure.
Nathan Bell:
<Laugh>. Well, there’s a few things, but I, I guess which ones do I, do I wanna share? Well, one thing that is unique, I dunno, maybe not so much, but my wife is actually A CFO, so we do have some interesting conversations. Now she’s on the government side, so she looks at things a little bit differently. But yeah, when you have two CFOs in the house, it makes for interesting dinner conversation. <Laugh>.
Glenn Hopper:
I was gonna say, just when, whenever a company comes over, they start looking at their watches and they’re, well, we better <laugh>.
Nathan Bell:
Try not to bore them. But yeah, I I think one of the other things that I, I always share in these types of questions is I was a pole vaulter in high school. In fact, I went to state for three years in a row at Pole vault.
Glenn Hopper:
Oh wow. I was actually, I was just at a pole vaulting, meet a friend of mine’s daughter is a D three pole vaulter in, in school. And I hadn’t, I haven’t watched pole vaulting since I ran track in high school. I don’t understand the physics behind it, but if you <laugh> anyone who could do that, that’s I’m, I’m very impressed with <laugh>.
Nathan Bell:
Yeah, it’s it’s definitely unique <laugh>. I think it was very difficult for my parents to watch every time I went up on that poll because so many other kids would fall back on their <laugh> Oh yeah. Path if they didn’t get enough momentum going up. Also, if I wanted to, to mention to the, to the listeners where they could find me
Glenn Hopper:
Yes, please, please do share that and we’ll put it in the show notes as well.
Nathan Bell:
Okay, great. Yeah, managing partner of vi consulting, va i consulting.com and we help folks solve these very problems we’re discussing.
Glenn Hopper:
Great. Great. Alright, so last question <laugh>, and I’m gonna give you an, I’m gonna, you’re gonna have an option here of whether to answer this or I’m gonna give you a secondary question. So the, the regular question is what is your favorite Excel function? I realize to you that may not be something that’s top of mind, so let me give you, and you can answer both if you want, but I’m gonna
Nathan Bell:
Say I still use Excel.
Glenn Hopper:
Yeah. Oh, we all do. I mean, do you I’m I’ll, I’ll be 90 years old making my shopping list or something. I’m still gonna default to making my list in Excel,
Nathan Bell:
<Laugh>. Yeah, absolutely. It’s, it’s never gonna go away. Never.
Glenn Hopper:
So alright, let me, let me throw ’em, but I’m, I want to hear your Excel, but I’m gonna throw the second question too. What is your favorite Excel function and what is your favorite Python library?
Nathan Bell:
Oh, geez,
Glenn Hopper:
<Laugh>. So you can pick one or you can answer both. No pressure
Nathan Bell:
Here. I mean, <laugh> like everybody else listening, if he look up, I don’t understand how you wouldn’t pick that if you were Excel user.
Glenn Hopper:
That was mine when I was a guest on the show. Yeah. With it.
Nathan Bell:
Okay. <laugh> <laugh>. I wouldn’t, I couldn’t live without that. And it, it’s funny, we try to recreate in our BI tools so much of what Excel does, but it just never quite seems to, to feel or fit the same way as much as we want it to.
Glenn Hopper:
Yeah. It’s just that, that old habit that you just know and it’s comfortable and <laugh>.
Nathan Bell:
Yeah. I like to tell people, it’s kind of like my scratch pad where you might have a notepad. I have Excel, like that’s where I do a lot of my thinking when I’m tackling problems.
Glenn Hopper:
Alright. Do you wanna, you wanna weigh in on the Python library or
Nathan Bell:
No, I’m gonna get back to you on that. Yeah. All right. <Laugh>. I will say this, I have been using Robo Susie a lot recently, or I believe it’s Susie, right? Is it? The robo. CFO.
Glenn Hopper:
Oh, the my my GPT. Yes. Rosie the Robo Rosie CFO. Yes. Yes, yes.
Nathan Bell:
Sorry, not Susie. Good Rosie. And it’s, it’s been quite handy.
Glenn Hopper:
Okay, Nathan, I really, really appreciated your time on the show today and your insights and we, we could keep going for another hour easily, I’m sure, but I think this is a good place to wrap. Yeah.
Nathan Bell:
Thank you. Appreciate it.
Glenn Hopper:
Thank you. Talk to you soon.