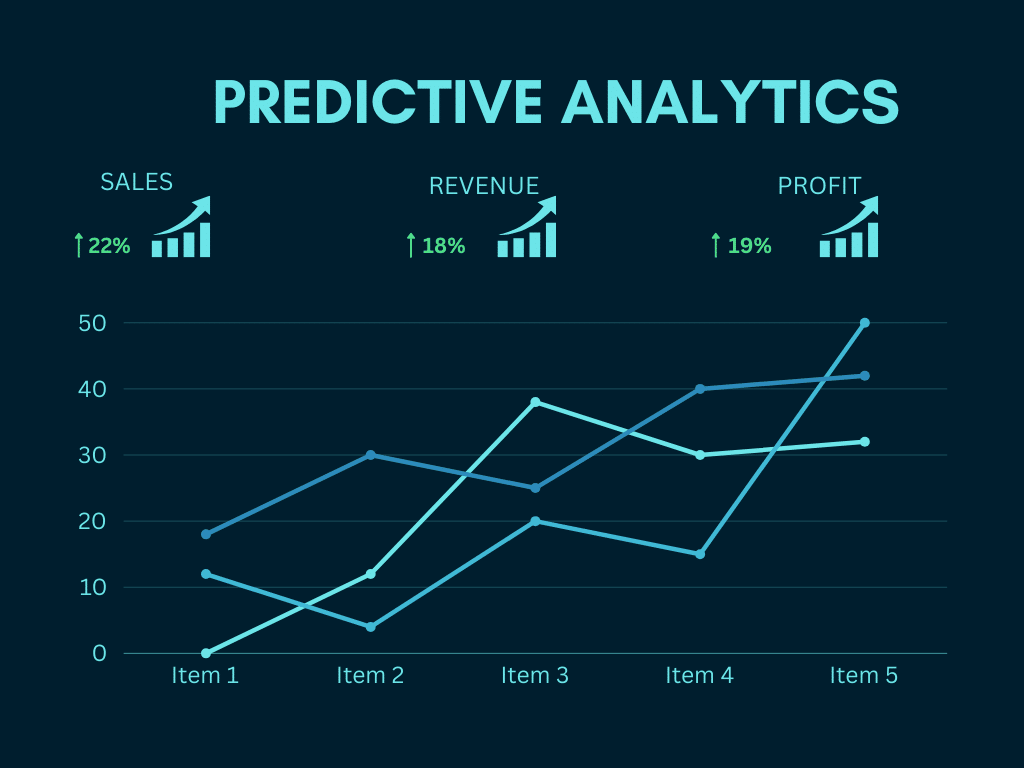
Predictive analytics in finance is a term used to describe a method of analyzing large amounts of data in order to identify trends and create more accurate forecasts. Predictive analytics uses different statistical methods to gather and analyze the data, and there are many different ways it can be done.
It can be overwhelming to understand all of the new technology that affects the finance department as there are new predictive analytics tools, AI software, and machine learning algorithms available every day.
So let’s go over everything you need to know about predictive analytics in finance, what it is, how it can be used, as well as a few case studies from companies that are already using it in the finance department.
What is predictive analytics?
Before we start talking about how predictive analytics in finance works let’s give a simple overview of what the term is:
Predictive analytics means using statistics and modeling techniques to determine future outcomes based on current and historical data.
In reality, predictive analytics is nothing new, but the technology is. It’s a form of data science – and mathematicians, businessmen, and scientists have been using these methods for centuries to try to predict future outcomes.
But with recent advances in technology, such as big data and machine learning, the amount, speed, and accuracy of the predictions have improved tremendously. We will be focusing on these new methods and tools.
3 types of predictive analytics
There are three main types of predictive models in the world of finance: classification, clustering, and time series models.
1) Classification Models
Classification models are the simplest model in predictive analytics because it classifies the data into a binary output – meaning there are only two options. For example, in finance, this model can divide investment opportunities into two groups – whether the shares will likely go up or not. Another way a company might use classification models is for determining whether a customer is likely to churn or not.
Classification models are most commonly used in things like risk evaluation, risk analysis, and fraud detection, but they can be used for most things in finance that involve a two-option answer.
2) Clustering Models
Clustering Models, also known as Outlier Models because they are used to identify outliers in a data set, are done by grouping different entities by identifying similarities and differences between them.
An example of outliers is a credit card company identifying out-of-the-ordinary credit card usage based on location or amount. An example of clustering would be grouping customers based on similarities and differences between them and using this information to create predictions and strategies for the different groups. Essentially both clustering and outliers do the same thing which is why they are in the same category.
3) Time series models
Time series models are the most complicated of the three as they combine different data inputs with time sets (monthly, quarterly, etc.) to create data patterns and trends. This is the one that is most commonly used by finance teams as it forecasts consumer behavior, inflation, changes in assets, and many other types of financial and consumer behavior.
Since budgeting and forecasting is rarely a yes or no question, and outliers are only a small part of finance teams’ responsibilities, time series models are the most common type of predictive analytics in finance.
How does Predictive Analytics help Finance?
Financial and FP&A analysts don’t just collect data and make reports; they are also there to forecast, predict, analyze, and help with decision making.
That’s where predictive analytics comes into play. The extra data and ability to predict and forecast can be a huge advantage over the competition and help propel the company forward.
Here are a few of the benefits of predictive analytics in finance:
- Better decision making – No matter what industry you are in or how much data there is, predictive analytics helps the company make better decisions on just about everything. It can help predict future consumer trends, market seasonality, and the ups and downs of the economy – all of which helps the company align with broader business goals.
- Risk Mitigation and Fraud Protection – Predictive analytics helps a business understand whether a loan or taking on a new customer is a good idea due to the risks involved. Furthermore it can help analyze fraud and catch it before it’s too late.
- Improve Customer Retention – By analyzing and understanding the customer profile in advance, businesses can keep their customers happy before they run into problems and potential churn.
- Revenue Growth – Whether you are an investment professional or a financial analyst helping your management team decide which product to invest in more, predictive models lead to more profitable strategies and revenue growth.
- Cash Flow Forecasting – A critical part of running a business is managing cash flows. You want to identify slow payers, solve systemic issues in cash flow, and improve receivable management. Data science and financial data analytics let you do just that.
Examples and case studies of predictive analytics in finance
Amazon Predicting Consumer Preferences and Behavior
It’s no secret that Amazon is really good at using predictive analytics to give consumers what they want. While most companies would not be able to pull this off on such a large scale, being the biggest e-commerce company in the world and having access to hundreds of millions of people’s shopping preferences and their data sure helps.
But Amazon is notoriously good at their product suggestions and anticipating what consumers want based on their preferences and past behavior. In fact Amazon’s analytics are so good they even toyed with the idea of “anticipatory shopping”. That means they ship items to closer locations, or even to your house, before you press “buy” based on your past behavior and other predictive analytics.
Pro Football Hall of Fame Using Predictive Analytics
The Pro Football Hall of Fame in Canton, Ohio was having difficulty combining all of their data into one system and coming up with actionable plans for the business. They started using Datarails FP&A and predictive analytics software, and were able to save many hours on the company’s monthly forecasting and budgeting process.
By using the predictive analytics function, the finance team could then create endless scenarios to highlight the different areas of the business. They could then easily show the key drivers to all the relevant teammates to help them make quicker and more informed decisions.
Future of Predictive analytics in finance
According to Statista, the predictive analytics market is expected to grow almost five-fold – from 5.29 billion USD in 2020 to 41.52 billion USD in 2028. But that doesn’t mean that everyone will be using a specific predictive analytics software.
As more and more companies are using software in the finance department (FP&A software, AR automation, accounting, etc.) predictive analytics will become more common within these tools.
In fact, many software solutions such as Datarails, Oracle, and SAP already have predictive analytics built into their tools and services. Finance teams are looking for ways to analyze and forecast all of their droves of data and predictive analytics will help them and the company make the most accurate and data driven decisions possible.
While predictive analytics might not stick out as much as other big changes in the world of finance software such as AI, it already is – and will continue – to make a big impact on finance.