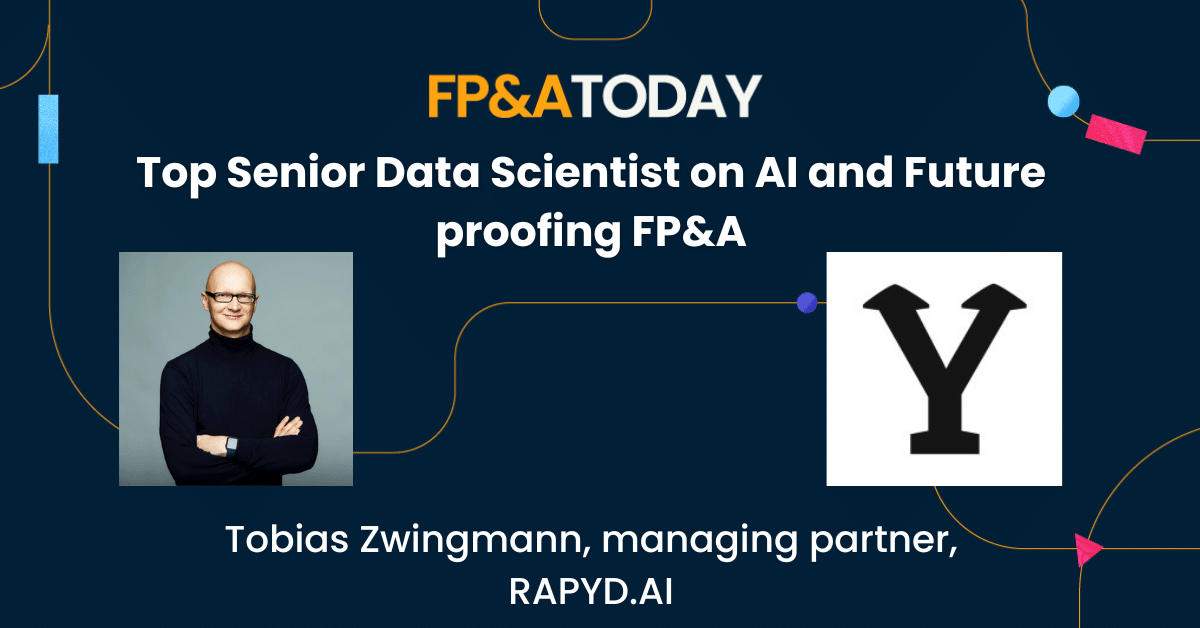
Tobias Zwingmann is an ex-Senior Data Scientist-turned managing partner of RAPYD.AI – which is empowering companies from B2B SaaS startups to leading financial institutions towards a unified AI strategy. His data insights are shared in his books including “AI-Powered Business Intelligence” (O’Reilly 2022) and “Augmented Analytics” (Co-author, O‘Reilly 2024) as well as weekly newsletter “The Augmented Advantage” read by 4,500+ business leaders from brands like Amazon, Mercedes-Benz, Gucci, and Santander.
In this episode:
- My rise in data science
- Did the latest changes to Co-Pilot actually make it good?
- What finance can learn from other departments using AI
- Consolidating reports and augmenting processes using AI
- Let’s talk forecasting and analytics and regression analysis
- Leveraging Excel and Power BI to enhance their data analysis capabilities
- Getting to “data progressive” and “data active” in your organization
- Augmented Analytics Explained
- Clean (but not tidy) data in finance
- Two ways to make data tidy in ChatGPT
Connect with Tobias Zwingmann on LinkedIn https://www.linkedin.com/in/tobias-zwingmann
Full Transcript
Glenn Hopper:
Welcome to FP&A Today, where we dive deep into the issues that matter most to finance professionals. I’m your host Glenn Hopper, and today we have an exciting episode lined up for you. Our guest is Tobias Zwingmann a seasoned data scientist, AI consultant, and author of two groundbreaking books, AI Powered Business Intelligence and Augmented Analytics. Tobias has over 15 years of experience in leveraging data analytics and AI to help businesses drive growth and innovation. Today we’ll be exploring how finance professionals can leverage AI to enhance their decision making, improve forecasts, and transform their organizations into insight-driven powerhouses. Whether you’re just starting your journey with AI or looking to deepen your expertise, this episode is packed with insights you won’t wanna miss. Let’s dive in. Tobias, welcome to the show.
Tobias Zwingmann:
Hi, Glenn. Hi. Thanks for having me.
Glenn Hopper:
Yeah, uh, super excited to have you on. I, um, I got your book actually for anyone watching the video. I’ve got it. Uh, here we go. It’s, uh, prominently placed, uh, <laugh> Awesome. This AI powered business intelligence book.
Tobias Zwingmann:
I appreciate it.
Glenn Hopper:
<laugh>. Yeah, so, uh, no, so huge fan of, of your work, and I really, you know, we, we talked about this before the show how, to me, how ingrained BI is to financial planning and analysis and how important it’s, so I, I love the work that you’re doing, and, um, I think it’s, it’s gonna really speak to our fp a professionals who are constantly getting better and better, like increasing their kind of, their data science and bi chops and, and finding value in doing that. So, really, really appreciate you coming on.
Tobias Zwingmann:
Yeah, really excited for this and, and talking about that.
Glenn Hopper:
So tell me, tell me about, um, your background, your journey from working in data science roles within corporations to, um, ultimately founding RAPYD.AI and becoming an AI consultant.
Tobias Zwingmann:
Yeah, so it’s actually been quite a long journey, I would say. So, um, like originally, like when I go back to my bachelor degree, I, I studied business administration, so I didn’t start out with like computer science or like AI or anything like that. Um, I was always kind of like technical though, like back in school, like building my own websites and tinkering with computers. But at some point I felt like I’ll have to do more business related stuff, <laugh>. Um, so, and I ended up actually like working in the corporate world for more than 10 years and in different roles and most prominently there data projects, analytics projects, and ultimately diet data science projects, which was like mainly due to the fact, fact that I did a master degree in IT management and learned our programming, which I found out was a high demand skill back then to know how to do R programming.
Um, so yeah, I worked in data science and mostly on, uh, like marketing and customer related tasks, like building shared prediction models or next best offer modeling for this whole, like, uh, customer sales marketing domain there, which was pretty good. But I also worked on, I would say more you would call these like digital projects. For example, back then I was working for an exhibition organizer and we developed new digital products that were able to track attendee behavior on physical event spaces. So that was pretty cool. We had really great projects there. Uh, also had like projects regarding data governance and data strategies, all that thing, these things. But then Covid happened. <laugh> Covid happened in 2020, and, uh, yeah, I was working for that exhibition organizer. And as you might know that, you know, during the pandemic, there are no exhibitions. So I found that this might be a really good opportunity to finally <laugh> make the jump, and I start my own company.
And that’s what I did back then In 2020 starting, uh, Rapyd AI together with a co-founder started out as a, as AI as a service business. Like we build an AI as a service platform where people can easily access AI services, but meanwhile it has more pivoted into an AI consulting business, um, because there’s such a high demand out there, not just for people to build their own use cases, but also to learn how to leverage AI for their business. And that’s where I think really like the demand is right now. People are excited about ai, which is great, but they are very often not really clear about how to use it most effectively and what to do with it, actually. And so, yeah, trying to help them figure this out. And that’s what I’m doing right now. <laugh>,
Glenn Hopper:
I definitely want to come back to the, uh, comment you made in, in your work in marketing, but uh, before I dive into that though, uh, you said something else that had me thinking about early on, looking back at the, the people who were able to use machine learning early on, it was the e-commerce companies and the SaaS company, the companies that were digital in nature and had access to all this data really before a lot of non-digital, you know, brick and mortar kind of companies did. But now, you know, obviously the, uh, those companies will continue and, uh, whether you’re a social media platform or, or e-commerce or something, you have so much data and that’s why you can have really good ai. But what we’re seeing now is with generative AI and everybody talking about it over the last year and a half or two years, even more so than we were before that for companies that are not in those super digital and, and maybe even, you know, just non-technical industries with where we are today, how do you see AI transforming businesses and can, do you have any examples from your consulting work around that or?
Tobias Zwingmann:
Yeah, so, you know, I think a lot of companies think they are, uh, that they are not ready for ai. That’s my experience. Like, they think, oh, we need to figure out like data governance and data strategy, and the whole data topic is so complicated and so on. And I would say at least until like two or three years ago, that was really the case. Like, if you did not have a good data, uh, structure or data infrastructure, it was really hard to do anything with ml or, you know, with ai. There were some AI as a service providers out there, which I also like introduced in my book where I like say, you can use AI, for example, to help you like fix data quality issues or to help you make sense of unstructured data and all these things. But it was still complicated through this.
And I wrote AI powered BI before ChatGPT was released then <laugh>, uh, even like back then, I was trying to emphasize the fact that you can use ai even though you are maybe not ready in terms of like data, uh, data maturity in, in the whole organization because there are so many opportunities. But especially since generative ai, I think there’s really no reason for any business not to figure out how to, uh, what I call augment or enhance processes with ai. Um, because it doesn’t matter if you are a SaaS business, if you are a high tech business, if you’re a brick and mortar business, they are typical things that are just recurring, uh, finance, for example, finance, you know, what I call money data. Every company has money data. They need to figure out like, you know, what’s the planning process like, what will the business be next year?
There are so many external factors, uh, but also marketing and sales, like the big second pillar, which in the end, you know, boils down to customer data. So every business has customer data. They want to figure out like what kind of products you offer to them. Like what’s the best pricing strategy, what’s kind of the best timing in order to release products, what’s the BA best way to to to communicate with customers? And with generative ai, there have been like huge opportunities that are, uh, that can be unlocked just by, um, by, by, by using it, for example, for for personal productivity reasons. I mean, there are different studies showing that people that use generative AI effectively can raise their productivity by something like 20 to 40% depending on which study you read, which is like massive <laugh>. If you’re running a big business and you have a 20% productivity increase, that’s, that’s huge.
Um, so I think there are different stages of use cases and there are those use cases where you need this super high level of data quality, but there are other use cases where it’s pretty easy just to get started. I mean, can be just as simple as like being faster reading and writing emails or, you know, being faster to generate reports or just being, uh, more, uh, more, more, more thoughtful about putting together a strategy paper or things like that. Right. Super, like, I wouldn’t say trivial, but like everyday tasks for every knowledge worker out there.
Glenn Hopper:
Yeah, and you know, I’ve heard, um, and Ethan Molik talks about this a a lot as, as well, that with generative ai, it’s not like, like if we were gonna roll out a machine learning platform and, and a, a big, uh, data tool in our, in our company, that would be done by, you know, it would be done from it with, you know, who, whoever the other involved parties are. And it would be mandated and, and dictated, you know, this is what we’re gonna use, this is how we’re gonna use it. But where people are seeing the best results with generative AI is when they put it out into the ha hands of the workers directly with the guardrails of, you know, don’t upload proprietary information here. Or knowing, okay, we’re on a, an open AI enterprise account, or we’re doing it in Azure, or whatever it is that they know, as long as they have guidelines for the employees to use and they give them some basic training and put the generative AI out there in front of them, people are finding ways <laugh> to use this that’s in their own benefits. So that’s, I think those efficiency gains are coming from companies that are actually giving employees the tools directly and not, not telling them use it to do this, but showing them how to use it and letting them figure it out themselves.
Tobias Zwingmann:
Yeah, yeah, absolutely. I, I completely agree with that. Um, and also the whole training aspect aspect around that. You can’t just roll it out and just say, Hey, we, we bought copilot licenses for all employees, you know, just, just go figure it out. And there might be some, like people who can figure it out and just because maybe they have used it in their private life, now they are using this for business as well, but most people won’t really care. Like they don’t, they don’t understand what to, what, what to do with it or, or maybe use it in a way that’s actually like counterproductive and, uh, like decreasing their output or generating worse outputs because they’re, uh, just prompting Chad GBT to say something like, you know, write a business plan for me for the next five years. And of course, of course it will produce something and it will write your business plan, but very often this business plan doesn’t make sense, so it’s not real aligned to your business.
So we have to be careful about that. But I agree like that this has been a huge democratization, uh, of AI also in terms of like taking AI and data away from the pure IT departments and just putting that more into the hands of business users, which, like, there, there have been trends before generative ai, but like generative AI has been like completely different new level in terms of accessibility for these, uh, for these products. Uh, like we see people interacting with AI that have never, like, had anything to do with data or machine learning before. So <laugh> and they are now Chad, GPD power users. <laugh>,
Glenn Hopper:
Yep, yep. <laugh>, because that’s, I mean, I think that’s, it’s, uh, which is great, by the way. It’s reinforcement learning, which is
Tobias Zwingmann:
Yeah, which is great. Yeah.
Glenn Hopper:
I’m dropping this on you when I have not even, uh, dug into this, but this morning I was seeing, um, a lot of updates on copilot. I don’t know if you’ve had a chance to look at anything yet, but I, you know, all the headlines are like, did copilot just get good? Um, I know there’s copilot pages, there’s a PowerPoint narrative builder.
Tobias Zwingmann:
Yeah, I’ve seen that. And honestly, I was surprised Microsoft is releasing that so quickly right now, I think they’re maybe on the pressure because like the previous copilot was not ideal to say the least. Uh, so there were high expectations, but honestly, I think like, I, I’m really giving Microsoft a second, uh, chance for this because I think it’s such a huge opportunity for AI augmentation because there are two main factors. The first factor is like, we have the, like most widespread analytics tool in the world, Excel has what, like 700 million users globally, something like, that’s by far the most popular analytics tool. And then we have Python, which is the, like, best understood language for ai. So every AI model that you have is like literally using like Python as their default way of like expressing themselves, uh, programmatically. So now these two things are coming together, native support for Python in Excel and copilot support in Excel, which is like, you know, a force like joining forces of like two super trends.
And if Microsoft really kills that one with their new COPI integration, which I still have to test out, but if they, if they make this really good, it will unlock so many like people to do so much more advanced stuff with their data because they can now literally ask for these things, and then the machine or Excel will be able to actually also do it before Excel was just able to tell you, okay, you need to do this and this and this, and, you know, run this formula and so on. But now with Python, like it’s a whole new level and, um, I’m really looking forward to that. But I, I, I remain a little bit skeptical because I’ve seen the previous co-pilot announcements, so let’s see how they do this one. Uh, but I would really appreciate it if it’s a amazing integration, but let’s see how that works out. But yeah, it’s like a total killer use case, like theoretically.
Glenn Hopper:
Yeah, it really is. And I, um, it really is. And I, uh, what I’ve thought from the beginning with generative AI is, you know, we’ve been talking about democratization of data for decades now, right? It’s like we need everybody, we need to tear down the silos and get everybody access to the data so they can use it. But what generative AI does, you know, the barrier to data science used to be you had to be able to do Python or be, you know, advanced BI tools and, and all that. And now if Microsoft can successfully integrate in copilot and let people just with natural language be able to access the Python and, and tied into Excel the tool that they’re already familiar with and already know, this gets really interesting. And so it’s not just democratization of data, it’s democratization of data science. Yeah.
Tobias Zwingmann:
And I, I tell you what, I think that’s my personal opinion. This will be a huge catalyst for data quality and also like the acceptance of data in general. Because what we saw before is that everyone was talking about like, we need higher data quality, we need better data, we need like less data silos and so on. But then people were like, okay, but why? What for? Well, because then you can use this spreadsheet and this BI tool and run this forecast and so on. But the problem is, and that’s also what I’m like discussing in the, uh, newest book, augmented analytics, very few people are actually doing that. So we assume that everyone in the company wants to open up an BI dashboard and like, see what’s the sales forecast for next week and so on. But turns out a lot of people don’t wanna do this <laugh>.
Um, but the, what a lot of people do wanna do in business is to make better decisions and to get questions answered. So if we find now that this new interaction layer where people can just ask questions, for example, in a teams chat to a chatbot or within Excel about questions they deeply care about, and they find out that everything is there except for the actual data that is now needed to answer this question, there’s a much higher pool, a much higher need in businesses in order to say, Hey, we need to figure out this data quality topic because I want to have the right answers in my chatbot. So I think that by the same way that, you know, AI got democratized and more people are using tools like chat, GPT, more people will now also like have a higher demand for, Hey, you know, we, I, I want to have this chat bot answer my question reliably, or I want to have this forecast being actually like valid because of the underlying data being correct. So by opening just that up to more people, I think this will give like the whole data <laugh> ecosystem, um, much higher pull effect. Uh, at least that’s what I’m expecting, but let’s see how, uh, how we speak in two years <laugh>.
Glenn Hopper:
Yeah, and you know, this goes back to what we, in it, it’s your mention of your start being in, in marketing and something we talked about before the show too, that marketing because of the data that they had, it seemed, to my mind, fp and a people have been, we’re the original business analysts, you know, going back <laugh> 20, you know, a million years ago when I started in finance, I mean, I, the business analysis part of what we do was very important, but then when machine learning came along, sales and marketing was using it way more than finance was. And I think it’s the nature of how much data they had and, and early on and also being, you know, the sexy department with the top line revenue and everything versus us just in the back office. But now, you know, kind of what we were talking about with copilot and with the, uh, democratization of, of data science. What do you think that finance professionals who are now, uh, getting more access to AI and, but what do you think finance professionals can learn from other departments like marketing or even operations who’s been using machine learning a lot, but when it comes to adopting AI and machine learning?
Tobias Zwingmann:
So two aspects, I I think from ai, what they can definitely learn is the whole like communication, storytelling aspect of it. Because what you can now do, like really easily with tools like chat, GBT is like, take your, your report, your forecast, your, uh, your, your, your, your, your, your dashboard and just turn it into like a comprehensive data story where you say, okay, this is the key inside, that’s what we need to do. It’s just 1, 2, 3, 4. Like, these are the arguments for this, which a lot of people like, kind of like struggle to do. Marketing is often the other way around. Like they have their opinion first, and then they collect data to support this opinion. So with the finance people, they often know the truth of, you know, what’s currently happening, but they need to find ways to communicate that effectively. That’s number one.
The second one is, uh, to just help them, like do lots of these tedious processing automatically or much faster. So for example, if you have to consolidate different reports into one big table, or if you have like messy data and you want to bring that into a tidy data format, these are all things where you, like, you could theoretically sit down and write an Excel macro or write, write some Python code that is kind of like automating that for you. But most people don’t wanna do this or they don’t have the skill to do it. And now with ai, they can just ask you to say, Hey, like, I have this report, that’s what I’m going to do. This is what’s happening every month. How can I do this? And then it will just give you some suggestions on how to automate this process and, uh, just make your work so much easier. And then ultimately the, uh, third aspect is of course, predictive analytics, which like, we can also talk separately about that, but I think this is a whole different field in terms of like, not only understanding what happened, but trying to predict and forecast what will happen in the future, not only considering the data that you have, but also external factors, external market trends, or also like actual data sets that you would not have access to normally as a, uh, controller or, uh, you know, fp and a professional.
Glenn Hopper:
Yeah. Let’s, let’s dig into that a little little bit more because on the predictive and prescriptive analytics, you know, that’s even small companies right now, and from a finance perspective, you’d say they have good descriptive analytics. They can look at the GL and they can say, you know, this is where we are. And this is, you can even say, you know, this is the trend we’re on and all that. And you can sort of do some, some basic forecasting, but the promise of machine learning and, and predictive analytics and ultimately prescriptive, but the promise is that you can use this historical information and like you said, start factoring in macroeconomic conditions and external factors and, you know, add new features to make the model more accurate. But for finance teams who are just starting to use AI machine learning, um, to enhance their forecasting accuracy, are there specific tools or techniques like kind of somebody that that’s first moving into this sort of predictive era? What, what advice would you have for them?
Tobias Zwingmann:
Let’s talk about forecasting. The actual tool that you are using is still the same. You’re doing a regression analyst, for example. Like it can come different variants or factors, but let’s say you’re trying to predict the, like, you know, budget or sales or whatever using a simple regression analysis. Maybe you are already doing that right now in Excel using the like advanced, uh, or data tools in there. Now what you can do with machine learning is you can still like double down on your regression analysis, but the way you get there is a different one. The paradigm you get there is a different one. Normally you would figure out, okay, what kind of like variables do I need to integrate in order to have good forecast and, you know, what are the parameters and so on to make it all kind of like statistically sign significant and minimize the p values and stuff with, um, a machine learning approach, you basically say, Hey, I don’t really, uh, prioritize explainability of my model so much, I just wanna make sure that the prediction is on point.
And please tell me what are the like three, four or five main factors that are driving this prediction. And for example, with Python and Excel, you now also have access to like the whole ecosystem of machine learning and Python, which is like SK learn psych, learn for like the main machine learning framework and Python, which is also like well understood by every large language more allowed there if you just ask it how to implement, you know, regression analysis using SK learning just the two lines of code. And it can help you do this. The good thing about this is that you can, a, get more data, like you can just like literally like make your table wider, get more variables in factor in more like external factors, for example, for different observations. And you will also be able to leverage, uh, tools from interpretable machine learning, which tells you which factors are driving your predictions.
Like, for example, if in plain English, what happens, like if, if this value goes up, which other values are also going up, like which kind of like effects or patterns do you see in your data? And this gives you a pretty good idea of, you know, what are the key drivers or the key influences in your data set for, you know, forecasting, uh, future trends or forecasting future future financial metrics. There are so many like fancy terms in there, and like it all looks so like, you know, kind of like scary for a lot of people, but it’s actually not that complicated. Not that complex, especially if you have the support or the help of an AI system built into Excel to do this. And I think there will be some really great insights that people can draw from that, not only for predicting future variables, but also from getting insights of, you know, what are actually the drivers here in my data set for certain, uh, financial metric, like, you know, sales or costs or whatever. Yeah.
Glenn Hopper:
Yeah. And it’s, um, you know, we’re, it’s, it’s gonna be really interesting to see how much Excel’s capabilities are expanded as more and more people, even if they don’t learn Python, if they’re able to do it through copilot and it’s happening under the hood, and then they don’t even know, you know, exactly how it’s working, it’s gonna open up a lot of possibilities in Excel. But one of the big things that you’ve talked about is Power bi, and I know a lot of fp and a departments have been using Power BI or Tableau and other reporting, you know, even, uh, data rails and other tools out there that give them, you know, flexibility in their, in their forecasting and, and the way they look at the, the data. Um, but if I, I guess looking now at the Microsoft universe, um, how, how can finance professionals leverage, you know, Excel and Power BI to enhance their data analysis capabilities?
Tobias Zwingmann:
In, in my opinion, it’s like you, you can use tools like Power BI from two different paradigms. And Paradigm number one is, which is most commonly used by, uh, financial departments, is for reporting and self-service BI purposes, which means you essentially like precalculate any possible combination in your metric, and you allow some slices and dices in your data so people can like drill down and get some insights that they need. This is the like go-to way where they hope that either themselves or someone else will do the actual work of like digging down and figuring out what are the like details here and what are the key insights. Paradigm number two, which I encourage people to try out more often, is just to load data into Power BI in essentially just one big table tidy data, which means every column is one observation. Uh, every column is one variable, and every row is one observation.
So for example, if you look at, you know, financial statements or if you look at different departments, you would have either different departments listed line by line and then different metrics, like for example, sales cost or whatever, uh, listed by, uh, by columns or for example, by products or customers, whatever. But that’s the kind of structure you would need. And now if you have that structure and you add more data, which is more variables to the table, it could be that you end up with a table that has 300 columns and 50,000 rows. And with modern tools like Power bi, this is like absolutely no problem to handle this amount of data. So you can just load it in there. And once you have that, you have now a table which is like super easily accessible for, uh, computers, for AI tools, but also for like just simple machine learning algorithms.
And if you do this, you can build in tools in Power bi, for example, like the key influencer tool or the Decomposition tree tool, which I explained in my book, AI Power bi, that allow you to drill down into your data automatically. So instead of like doing all the slices and dices by yourself, you will just tell the algorithm, or you would just tell the system this case Power bi, Hey, this is my data, I’m interested in, for example, the sales metric. If this goes up, which factors are influencing this variable? And if we just run the calculations and show you the top 10 or top five metrics that are influencing this variable, uh, or for example, take the decomposition tree where you say, okay, this is my, uh, high level, uh, my high level KPI, for example, total sum of revenue. And then you want to break that up by, by different contributing factors.
But you want to do it in a way where the most important factors are split at the first level. So for example, the first could be by product, by region, or by country or by, uh, sales department or whatever, but the AI will figure out what is the best split to make at the beginning to have these greedy splits to see, okay, who’s driving most of what, and then go deeper and deeper, totally automatically. And this is a feature which has been in Power BI for, I don’t know, like five years or something like that. It’s like been there for a very long time, but people are not really using it because mostly they are still operating in that, you know, paradigm, one mindset where they think, okay, we need to deliver all the knobs and buttons so people can do these calculations by themselves instead of thinking, Hey, maybe we just, just just just just try to integrate, um, our different data sets into one big table and then allow Power BI to get these insights, uh, to help them get these insights much faster. Uh, so that’s what I, uh, do, do definitely recommend. I, I don’t know if Tableau has a sim similar functionality, to be honest. Maybe they have, but Power BI definitely is a pretty good tool for doing this.
Glenn Hopper:
To me, when I get all the data consolidated like that, I like to start looking for correlations, <laugh>. Yeah. And not that, not that I’m jumping to, that’s automatically causation, but you know, if this isn’t an academic research paper, this is trying to find some insight into your business. And if you, once you sort of have that view of everything you’re going through, you’re looking for these correlations and trying to find, you know, new information about it. And I think is, um, I don’t know, kind of a tangent there, but <laugh>, um, that it’s just a, as you consolidate, um, all this information, it’s easier to start trying to find those correlations.
Tobias Zwingmann:
Yeah, absolutely. And like in the end, very often you are not looking for these like 0.2 percentage, increasing point, but you’re looking for like the high level in size. Like, you know, what’s the main, what’s the top three drivers that are contributing that our revenue will be declining in the next three years? Like, this is like super important business questions and uh, like tools like this can help you figure this out, this out, like in an instant given you have the data and if you have it in the right format. But I think especially in fp and a, that there’s such a big opportunity for, uh, getting these insights just from the, the project that I have worked in, uh, where most people stumble upon is that they, they try to kind of like aggregate data first and then load it into DBI tool because they are afraid the BI tool can’t handle all the data.
Whereas what you actually need to do is like load data in, in a very granular form, like ideally like every sale by every customer, or at least, you know, uh, aggregated by a weekly sale or daily sale or depending on what the size of your business is, but at least getting to a level of granularity where you really like maxing out the possibilities of your BI tool. Uh, because like what I see very often is that these BI tools are like not really operating at their maximum level of capacity. They are sometimes they’re working at their maximum level of capacity in terms of like understanding, like from a people or human point of view, understanding the complexity of the data set. Because what people have will have in the end is like a hundred different tables with different aggregates. You have an aggregate table for this value, an aggregate table for this value, you need to figure out how these things correlate or can be brought together.
And this is what really makes it very, very complex. So this is, I think, a different paradigm in terms of how to think about data and how to approach data for loading it in to your BI tool. And again, this is not how you would bring that out as a self-service, BI solution tool, like all your users. This is more something like where you as a, like, you know, solo analyst or business analyst sit down and want to get the insights for certain questions fast. And this is where you can leverage these BI tools for, especially with Power BI desktop, which like just runs on your computer, and if you have a, like, pretty decent, uh, modeling computer, uh, it’ll have a good amount of storage to to process that data.
Glenn Hopper:
Yeah. If you aggregate everything, you kind of, I guess you <laugh> you lose the trees for the forest when you aggregate everything and you’re just working on, on the aggregated data versus I think maybe the mentality of thinking of if I’m using something, you know, very specific, a a random forest, a decision tree or you know, gradient boost or whatever, I’ve got this sort of explainable model. But you know, and this, you could go even deeper and say, well, if we were able to use deep learning, the more data we, we don’t know what features are important. So we, you know, if we, the more data, the more granular data we have, we load that into the model. And by, and by not collapsing it into these augmented groups, you can actually find real information.
Tobias Zwingmann:
Yeah. And, and in the end, you know, these are the, these are all the things that you can do. But, but these things, of course all come with a cost because like, there’s cost of maintaining this data. There’s cost of moving this data, there’s cost of just like making this data accessible to, to the analyst. And in the end, you need to have like a good like use case or business case for like use using this data or for working with this data. If there are more users because of the democratization of AI and the like easier interface of like running these queries or just asking a question, then there will be also a higher potential for unlocking or for, for bringing this data in because there will be more people benefiting from it. Simple example, currently without generative ai, we need to build this predictive model and put it into the dashboard to have these forecasts and so on, which like ultimately limits the scope of this tool to people working in the BI tool.
But if we now take that for example, and, uh, and, and, and integrate it into a teams chatbot where you can just ask, Hey, what’s my sales forecast for next year? And then the next question will be, oh, why is that so bad actually? And just gives you the answers and it gives you the answer based on the exact same analysis that you would do in the BI tool, but just it’s running that under the hood and just formatting that information in a way it gets, uh, transmitted or communicated to you in a more natural way. Uh, that’s such a big breakthrough, and I think we are not really leveraging that yet, but if we do it in that way, I think like people working with like data and data analysts will have such higher leverage inside their organization ’cause just more, uh, more, more easily accessible to everyone.
Glenn Hopper:
FP and a today is brought to you by Data Rails. The world’s number one fp and a solution data rails is the artificial intelligence powered financial planning and analysis platform built for Excel users. That’s right, you can stay in Excel, but instead of facing hell for every budget month end close or forecast, you can enjoy a paradise of data consolidation, advanced visualization reporting and AI capabilities, plus game changing insights, giving you instant answers and your story created in seconds. Find out why more than a thousand finance teams use data Rails to uncover their company’s real story. Don’t replace Excel, embrace Excel, learn more@datarails.com to be able to get to that point.
You really have to kind of reskill and upskill everybody across the organization. And I know you’ve talked about the need for more data literacy within organizations, and I’m wondering for, for companies or for departments who feel like, you know, they’ve got a little bit of a gap to get across there, what steps would you say that companies or individuals could take to close that gap and ensure that, um, they’re kind of, that they’ve positioned themselves to use these AI tools?
Tobias Zwingmann:
Yeah, so there’s like, there are four stages where a company can, can, can be in, um, and the first stage is really you haven’t, like, your company is not doing anything with data at all. Like, you know, you’re just like sleeping over the whole data thing, which is like not so many people out there. The, uh, second stage where most or a lot of companies are already in is, um, what I call data active. Like data active, um, means they are using data to, uh, make decisions and to like, you know, influence their reporting or to make better business decisions. So they’re actually actively trying to seek data in order to drive their business forward. And a lot of people are there every time you have like an internal BI tool or you’re doing some tr like some trends analysis or, so that’s basically the data active stage.
The next stage is data, progressive data Progressive means you’re more doing of more doing these like predict analytic things. Maybe you have a data center of, uh, analytics center of excellence in your organizations and to get to this level, like data progressive, you can use upskilling and like getting more people data literate because this essentially means that you have a certain number of people in your organization, maybe 5%, maybe 10% if you’re lucky, maybe 20 or 25% of quote unquote data professionals or highly data iterate people that are, I ideally spread across different departments that can help these departments to like get better insights, to make faster decisions, better decisions, and so on and so forth. But the ultimate level, which is like data fluent, which means like every business process in your organization is infused by insights and uses data in order to get this competitive edge.
I don’t think you can get to this stage with pure education and pure like data literacy because this involves everyone in the organization to become more like aware and, and more thoughtful about data. It turns out, at least that’s what I’ve seen. And also working with like larger organizations that are like in this journey for the past, like almost decade, not everyone in an organization wants to do this. Like there are so many people that really don’t care about data. They, they don’t wanna open a spreadsheet. They think that even an Excel table is just like too much hassle and even thinking about like running their own forecast, it’s just like too much. So what we need to do in order to reach these like group or this group of group of people is not to try them upskill more and more. We can upskill them to a certain level in terms of like, that data is critical and relevant and you have to be like thoughtful about your decision making and so on and so forth, but not on the tooling side for these people.
We need to bring the whole tooling side closer into their work in their workflows. And this is what augmented analytics is all about, trying to integrate and infuse insights into the work that they are doing on a daily basis. Simple example, if you are a sales, uh, sales rep and you want to know which customer to call first because maybe you have a customer list of like a hundred customers and who are you going to call first? You are not that logging in to BI system to figure out what’s the highest opportunity right now. Uh, what you do is maybe you log in into your CRM system and you want to have this insight into your CRM system, ideally in your dashboard, personalized to your specific account or to your specific, um, user profile. And this is where you can bring in these, um, augmented analytics tools to bring these insights to users so they don’t have to go somewhere to get the information, but this information is just like neatly integrated into the workflows that people are doing.
Or for example, it can also be more for, uh, in the fp and a fp and a well, for example, imagine you have a CFO who is thinking about, okay, what could be the, like, you know, uh, what could be different scenarios for our top level growth for next year? Very often they don’t want to go somewhere to a like different tool or to a Jupyter Notebook that is running a forecast analysis or so on that they just want to have this solved in the same tool, on the same interface where they are just right now consuming this report. And this might be Excel, this might be Excel. And so we need to bring these advanced insights or advanced analytics and these insights into this Excel spreadsheets. And this is why I’m so excited about like the Python integration and co-pilot and all these things because it allows us to like tap into this really well proven ecosystem with, uh, very advanced technologies and very advanced advanced models.
But here’s the thing, like you can’t really leapfrog or shortcut to this last level. You need to have a maturity, which is at least data progressive or data active that has guardrails around data governance, that has some guardrails around a data, uh, data analytics center of excellence that is monitoring, like what is being done in the organization. That’s only the final stage, but you have to go through these stages before and make sure that you have like all the, all the groundwork in place because otherwise what you will do is you will infuse wrong or, uh, potentially misleading insights into the workflows. And then you don’t really have a, uh, net positive, but a net negative impact on your business. And you don’t wanna have that.
Glenn Hopper:
Let’s dive in because your second book is on augmented analytics. So I wanna dive in and and unpack that a little more. So what does augmented look analytics look like? I guess one to the person who’s the, the beneficiary of it and two to the person who’s putting it together. When, when you talk about, so you’ve got a a whole book on it. So what is the, what’s the idea of augmented analytics and how it, how a company could move towards applying?
Tobias Zwingmann:
Yeah, so augmented analytics, and I have to say that because this is actually like the whole book is actually a, uh, big learning story from, uh, my co-author Will Viva, who has been driving an analytics transformation in a very large insurance company here in, uh, Europe over the past couple of years. And where they did exactly, uh, that they face this ceiling of sync. We can’t upskill everyone, but we still need to, uh, make sure that everyone in the organization is like using these insights in order to make better decisions because especially in like insurance or financial businesses, it’s all about like interpreting and making sense of data the right way. Like that’s the core business model, actually risk management, risk assessment, making sure that you have the right pricing and everything. So there’s lots of, uh, like data involved. And the, uh, big learning that, uh, that, that we came up with was to say, okay, we need to give people an analytical leverage.
What do we mean by that? Analytical leverage means that people are able to draw insights from data without being qualified to do so. So for example, they get a certain, uh, they get a certain, uh, they, they are with, they are within a certain workflow and they just get context relevant insights for making a, uh, business decision. And there are so many places and business where you have these like context relevant augmentations where either by looking at different examples or by, uh, looking at certain forecasts and giving an explanation of why that forecast might happen, and just bringing these in into the actual workflows where, where people are in, uh, chatbots are a different example where we say like, you can augment, uh, Microsoft team channels, for example, with a data analyst chatbot where people can just ask this chatbot while they are, for example, in the conversation with a colleague over a team, over a team meeting instead of like leaving the meeting and then logging into a BI tool, which no one is ever doing. Uh, but instead relying on some gut feeling you could just bring in the chatbot and just ask this chatbot some targeted question questions, right? Within the conversations that you’re currently having with your either department head or with some other stakeholder. Just get the insights from there. And so these are all examples of, um, this spirit of augmentation. And again, it’s all about bringing people this analytical leverage to ultimately make better decisions, uh, and build better business outcomes.
Glenn Hopper:
That’s really important to me to, as a message. So the past several months, I’ve probably had, I, I’ve had several, uh, data based guests on to the point where some of our listeners are probably saying, am I listening to data analytics today or fp and a today <laugh>, but it’s, this is very important to me and we, and fp a remains crucial and we’ll, we’ll keep talking about that and all the components from, from the reporting to the, the story and everything we do around it. But with generative AI and with increased automation, I think where fp and a professionals we used and early in my career, you know, some of my proudest moments were these super complicated models I built in Excel. It’s like, look at all this look at, you know, in just this massive spreadsheet that, uh, you know, took a decoder wheel to understand how everything happened, but you know, these very complex models.
But as that gets more and more automated the way that we are gonna add value, like you said, if you have a generative AI tool and you can just go into Microsoft Teams and ask it a question just like you maybe used to, it’s the same question. Maybe a couple years ago you would’ve asked your head of fp and a Yeah, now you can just ask a chat bot. Yeah, well, how is that head of fp and a gonna provide value? And I think it’s gonna be by learning to harness and use that data more and more and to be fully integrated into it. So I would say whether I’m, you know, someone who’s been an fp and a analyst for, um, you know, a, a long time or if I’m very early in my career, if I’ve just been, I am an Excel warrior and I can do anything in Excel.
And now <laugh> though, you know, looking at the potential and the increased amount of data and kind of how more and more people, it won’t be a superpower when a brand new Excel user can go in and in natural language ask Excel to do something that you used to do. So, you know, I think by integrating and, and kind of re-skilling and, and broadening that horizon into something like Power bi, I mean, what do you think for someone who is that they’re super high level at Excel, but they wanna make that transition and broaden to Power bi, how can they, how can they sort of make this transition in the smoothest way to expand their, their skillset?
Tobias Zwingmann:
You mean like transitioning from Excel to, to Power bi, uh,
Glenn Hopper:
And be, yeah, and, and I don’t mean one at the expense of the other, but being able to sort of integrate their work into bring Power BI into their workflow.
Tobias Zwingmann:
I would say the, like the most important thing right now is to, uh, will be to understand the data structure that Power BI needs to be most effective. And I, I, I briefly discussed that before, this is like the tidy data format and maybe we can put a link or so in the show notes because lots of data sets that I see in Excel are not tidy. They might be clean, but they’re not tidy in this like data analytics sense because maybe they have merged table cells or, you know, there’s some text going like vertically somewhere. So like, these are all things that are like super hard for computers and especially Power BI to make sense of what you want to have is a flat table or multiple flat tables where every row is one observation and every variable is, uh, one column and every cell has one single value.
If this is the case, then this is tidy data and this makes it super easy for Power BI or any other analytics tool, Python or whatever to work with this data. And I think for lots of people, the big aha moment will be to realize how to bring their like untidy data into this tidy format. And for this actually, power Query is actually the, uh, big unlock because it helps you to like run these data transformations and, for example, have this kind of messy format, but always bring it into this tidy format automatically and like just rerun the whole pipeline, everything this gets updated. Um, because once you have your data in a flat table, just as simple as saving it to an actual file and just opening this actual file with Microsoft Power bi, that’s it. And then you just use some of these prebuilt tools, like for example, the key influencer tool, or you just use the build in co-pilot to ask it questions.
There’s the natural language q and a tool inside Power BI to like ask questions right away to your data. So once you have your clean data in tidy shape, everything is good. What I’ve seen mostly in, um, like in the financial domain is that data is pretty clean <laugh> because like people really care about this data to be accurate, but it’s not tidy, it’s not in the right format for analytics to really make sense of. And what they, what people often do is just to make a copy of this data and then another copy, and then, you know, look at this from this angle and from that angle, maybe pivot it here and there just to answer different questions. But what you act, what you want to do is to like bring it into this tidy format and then use that as your central repository to draw any analytical query you have to this special table.
And I think this is the, this is very often the missing puzzle piece of like bringing Excel tables to Power bi because what a lot of people are doing is they just take their Excel reporting file and then upload this to Power bi or even upload it to chat GPT, and then they are wondering why it can’t work, why it can’t work with this data, or why the results are just so underwhelming. Well, because your data is not in the right shape. Yeah. And this is, uh, and, and I, and I think like it only makes sense if you look at how data, especially in fp and a is supposed to look like in the end because you have, like, if you look at the balance sheet, right, that’s kind of like the single source of truth. And then from there you just go deeper and deeper into all these different realms.
And also if you think about business cases and scenario planning and so on, like these are not formats that are made for like machine or algorithmic based data analysis. So you have to bring it to this format. Uh, so yeah, I definitely recommend learning, uh, power Query in order to have these transformations. Correct. And for this, actually chat, GPT can like be super helpful. For example, uh, that’s also one thing that I teach in my courses is like if you have a, if you have an Excel spreadsheet, just take a screenshot of that spreadsheet, paste the screenshot into chat GPT and ask it, how can I make this data tidy? Using Power Query, you will get step by step process of what to do and which step to do in Power Query. In order to make this data tidy. You don’t actually have to understand to a full level what tidy data is, but at least by knowing that you need to bring it into this format, and this is the key word to tell JGBT, uh, to, to give you these instructions, uh, you’ll, you will be far ahead.
Glenn Hopper:
Yeah, I’ve, I’ve actually, uh, found some super quick things, ways to use chat GPT for data cleaning, and here’s, this is a, a super simple one, but it, it’s funny. So first off, you know, if you’re just uploading a Microsoft Excel, um, document into chat GPT, it really would much prefer a a CS V file that doesn’t have all the yeah. Meta information that doesn’t have all the meta information and all that. But then, you know, it’s, you were talking earlier about, um, columns being your features and rows being your observations. Well, if you think about in, in finance, and so you know, all the financial statements are the rows are your accounts, so those are actually the features and then the dates going across the X axis. Yeah, that’s your observation. So even just having chat TPT transpose that and flip it to a way that makes more sense for a machine learning model. And that’s something it can do really quick. And it can do things like, uh, you know, if, if you’re gonna be doing some kind of predictive, uh, information or whatever, if you have categorical variables and, and numbers that’ll make sure everything’s in the right format. So chat GBT has saved a bunch of time for that, that data cleanup. And I would imagine as you get that into Power BI or any of the tools, that’s, but I guess with Power Query, you could do the same thing very quickly.
Tobias Zwingmann:
Yeah. But I think, like, you know what, what, what most people do is they, they, they, they try to fix this like messed up data structure in Power BI through just different reports and like by trying to figure out like what is the measure, what is the actual, uh, dimension here, and just like turning a measure into a dimension and then just messing things up and it gets super complicated. Uh, so just try to load it into the right format and then just run this whole, uh, run this whole thing. And then, then the next level would be like, once you have a tidy data set or a tidy table, just take another tidy table and figure out what’s the link between those two. Like, this could be a date stamp or this could be a customer ID, or this could be any other point of reference, uh, because this would be like, you know, a data model in terms of primary and foreign key.
But this is essentially the connection where you can, uh, you know, just, just combine both data sets without actually merging them in one big table. Uh, you can just say, okay, this is like my customer table and this is my, uh, like sales table. And once, like, there’s an ID here and there’s an ID there, and then the power bi, you can just define it as a relationship. You don’t actually need to bring it to one table, but this is how you like, step by step, build your analytical data set by figuring out like what’s the most important thing that I’m looking at here. For example, like could be, uh, could be sales transactions, could be cost, and it could, could be cost distributed or different departments, whatever. And then, and you figure, and then you, uh, you think about for every row, what’s the different or what’s the other dimension, the other column I want to see in there. And if you have that in the roof table, you just need to figure out what’s the missing link between those two. And that’s how you like step by step add complexity to it and like also unlock deeper insights if there are deeper insights. Sometimes you figure out that, you know, just by adding more data, there are actually more insights to it that can also happen. Um, so yeah,
Glenn Hopper:
I know we’re running, running long on time, but I do want to hit one more thing before we get to kind of the, the personal and fun questions. Um, I, you know, everything that you’re working on in the analytics space and books and, and teaching around it and the, and the consulting that you’re doing, I mean, we’re seeing that companies across the board, everything is getting more data driven. Certainly finance is, is getting more data driven. And as we are looking at making, you know, and, and also more is getting automated now with generative ai, it’s gonna be quicker to do these reports if we’re not spending all of our time just putting together these models and these reports and, you know, doing our, our our presentations. Where is finance gonna add the value? And I guess what I mean is what skills do fp and a folks need to really focus on right now as more and more as automated? And we’re relying more and more on data. How, how can you kind of future proof yourself or, and put yourself in a position where you’re riding this wave rather than, you know, paddling behind trying to catch up.
Tobias Zwingmann:
It sounds stupid, but you have to get hands on with ai. And the reason I’m saying this is right now, AI has, you know, what’s often called also by eol, uh, a, a eject frontier, which means there are certain things that AI can do exceptionally well. Like, for example, like making a prediction based on a historical data set with like 5,000 columns and a hundred thousand rows and figure out what’s the most important thing. Like it can do that in a fraction of seconds. But there are other things where it really struggles to find an answer, things that we would normally think, well, it’s actually so, so simple to give that question. Even Chad, GPT, like until for, uh, until like two weeks or so struggled with like doing very basic mathematical, uh, aari math, uh, a arid mathic or very basic, uh, mathematical operations.
And this is just because of the way of these, how, how these models work. But the point is that just looking at it like from an external point of view, you would say, wait, if you do these advanced forecastings, like why can’t you do basic mathematics in high school grade? Right. It doesn’t make sense. So, and and it’s quite hard to explain why this is the case. There are reasons for it, but it’s like it takes some time to explain the best thing, really to figure out how it works and what does not work. And what works is really just to get hands on and try it out because this frontier, this jagged frontier is also, is also moving. You know, we had just saw a recent release by open AI where we now have like higher mathematic capabilities in these models and who knows what’s coming in six months.
So you need to be kind of like on the pulse and figure out regarding the tasks and the workflows that are relevant for your daily job. Like which of these are inside frontier of ai? Because that areas where you need to leverage AI in order to do this for you or do it together with you. And what are still areas that are kind of considered outside of the frontier of AI where you need to still like double down on your own like human reasoning and your own human perception of these things to drive the needle. This is how you really Yeah. Can, can future proof yourself. Um, I don’t really believe in like enrolling like in like super long bootcamps and studies and, and and all that because like you will learn a ton of things. I mean, it’s great for learning purposes and if you want to like pivot careers and so like, you know, that’s totally fine.
But just, I think for most people it’s very hard in the end to say, okay, what will make an impact on me and what won’t, just the best way is to reflect what are you doing every day? You know, what’s your typical working day looks like? You know, what are things that are coming in, what are things that are super annoying? And then just trying out how AI can actually help you here with these things. Um, and figure out like how does the frontier look like for your current job? And make no mistakes. Make no mistake there, there are jobs where literally all of the tasks of what people do are inside the AI frontier. I recently read an article of, uh, professional translators in the European urine, uh, EU parliament where like they don’t worry about their, uh, jobs because like they’re employed by the eu.
Like they, they can literally <laugh> retire there their life. They, so it’s not about like job security, but they are literally saying, well, you know, we are pretty sure like in four years from now, our job will be non-existent anymore because translation is inside the frontier of ai. And especially with regards to like all the things that are being discussed, like on the political level because like, these are terminologies that are well known and like AI is pretty good at, at solving that because it has been trained on like 20 years of translation material from all these folks. Um, so these are like completely inside the frontier and like it’ll be clear that their job might not be existed like five years from now. Uh, for fp and a, I think like there’s more nuance to it, <laugh>, there might be parts that are inside the frontier, but there are parts which are like outside the frontier.
Uh, and there’s no like way to answer that in a single sentence. What is inside what is outside. You have to figure it out for yourself. Uh, and I think this is why getting hands-on is so important because it, the experience just adds up over time. You start learning and then you start picking up and figuring out, oh well this works. Oh, this works if I do it like this, and so on and so forth. So it has to be this like continuous journey. Uh, so just allocating sometime one hour, two hours every week, you know, just dabbling around seeing how these things work and then also integrating that in your daily workflows, if that’s possible. Be ideal <laugh>.
Glenn Hopper:
Yeah. Yeah. And and the added benefit of that is as you start integrating it and learning more, that becomes your superpower and you start to be able to be more efficient and have better models and all that. So you’re, you know, you’re, you’re keeping up with the technology but you’re also making yourself more valuable by being able to use it.
Tobias Zwingmann:
Absolutely. Yeah. A hundred percent. Yeah.
Glenn Hopper:
Well we are getting close to end of time, but I always, we, we always wrap up with a, a couple of questions ’cause we just dove straight into our nerd stuff and, uh, <laugh> and got into all the analytics and everything. But before we, uh, let you off the hook here, um, one question we always love to ask the guest is, what’s something that not many people know about you, kind of some, some inside baseball in your life? <laugh>.
Tobias Zwingmann:
I know I typically don’t share much personal things, uh, also online. Um, but like what I do in my free time, uh, whenever I’m not on the computer and like, you know, doing all the AI and data analytics, it’s just like basically spending time with my family. I have three kids under the age of 10 and like lots of people ask me how I can still, you know, stay sane like with running a business and having family life and everything together. ’cause if you have these, uh, nights and I like, you know, father of young one will know that where you work, where you sleep like four hours or five hours if you’re lucky, you know, you can really get crazy, uh, <laugh>. So I’m behind that stage right now. But yeah, that, that’s something that I still need to juggle. And actually I was thinking about also like writing a little bit more about how it is to be like, you know, a parent and working full time and I’m doing all these things and also trying to keep up with everything that’s coming here.
Because if you think about it, like there are so many questions attached to that. Like, you know, like my kids are now going to school, they are learning handwriting and you know, calculating in their head and everything like that. And I’m like, oh gosh, should I intervene or should I just let everything happen and just watch them know for the best in the end? Because honestly, I mean, I I tell you, I I do think our education system is absolutely, it’s not prepared for what’s coming. I mean, there will be a big impact in the next two years, but the impact for the next 10 years, it’s just like no one, no one’s good. No, no, no one’s is prepared for that. And so I’m really wondering, okay, well what does the future look like? Especially for, you know, the, the young generation right now. Um, yeah. So yeah, that’s something that not a lot of people, uh, know about me. <laugh>, I’m thinking about that quite often actually.
Glenn Hopper:
Yeah. And on the education front, what happens when you can, especially at, at the sort of primary and, and and high school level when you can completely outsource thought chat. GT four oh was, they said, you know, the equivalent of a, a smart high schooler.
Tobias Zwingmann:
Yeah.
Glenn Hopper:
And then, you know, it’s, it’s kind of moving up the chain now, but what does that do to education when, you know, every bit of your homework you could outsource to chat GPT or whatever the latest LLM is. Yeah. So that’s, that’s a whole other episode and we are late in it, so I guess I should get <laugh>, but I I, I hear you on that and it’s gonna be interesting to see the transformation there. Um, okay. Everybody’s favorite question we always have to ask. What is your favorite Excel function and why? <laugh>
Tobias Zwingmann:
<laugh>. I think my new favorite Excel function will be the Python function and Excel from me run Python code. <laugh>.
Glenn Hopper:
Yeah, <laugh>. That’s, you know what’s funny is as that, as co-pilot and Python is more and more integrated, I it’s gonna be like, you know, we used to remember everybody’s phone numbers and uh, and you know, and like even memorizing passwords for our logins and everything, I feel like at some point we’re gonna forget the names of these functions ’cause we’re just, it’s gonna be whatever we call ’em in natural language.
Tobias Zwingmann:
Yeah. <laugh>.
Glenn Hopper:
Absolutely. Yeah. Yeah. And then Python’s gonna go do it. So nested ifs and index match and all that. Oh, well don’t even remember what that’s called. <laugh>. Okay. Last question. Um, how can our listeners connect with you? How can, you know, find out more about your books and I know you’ve got another one coming out. What’s the best way for for people to reach out and stay in touch?
Tobias Zwingmann:
Yeah, I think best way probably is to connect with me on LinkedIn. I’m pretty active there. Uh, but also subscribe to my newsletter, uh, to be a sigma.com. You will be re re redirected to my newsletter. So feel free to subscribe that I share some AI rated insights every week on Friday. Uh, so yeah, sign up if you like and to keep in touch. There will be Great.
Glenn Hopper:
Tobias, thank you so much. Really enjoyed having you on the show.
Tobias Zwingmann:
Thank you so much for having me. It was a great pleasure.